Open Access
ARTICLE
Intelligent Deep Learning Based Multi-Retinal Disease Diagnosis and Classification Framework
1 College of Computer Engineering and Sciences, Prince Sattam bin Abdulaziz University, Al-Kharj, 16278, Saudi Arabia
2 Saveetha School of Engineering, Saveetha Institute of Medical and Technical Sciences, Saveetha Nagar, Thandalam, Chennai, 602105, India
3 Department of Medical Equipment Technology, College of Applied Medical Sciences, Majmaah University, Al Majmaah, 11952, Saudi Arabia
4 Saveetha School of Engineering, Saveetha Institute of Medical and Technical Sciences, Chennai, 602105, India
5 Department of Electronics and Communication Engineering, College of Engineering & Technology, SRM Institute of Science and Technology, Vadapalani Campus, Chennai, 600026, India
6 Department of Applied Data Science, Noroff University College, Kristiansand, 4608, Norway
7 Department of Computer Techniques Engineering, Al-Mustaqbal University College, Hillah, Babil, 51001, Iraq
8 Department of Computer Science and Engineering, Soonchunhyang University, Asan, 31538, Korea
* Corresponding Author: Yunyoung Nam. Email:
Computers, Materials & Continua 2022, 73(3), 5543-5557. https://doi.org/10.32604/cmc.2022.023919
Received 26 September 2021; Accepted 01 December 2021; Issue published 28 July 2022
Abstract
In past decades, retinal diseases have become more common and affect people of all age grounds over the globe. For examining retinal eye disease, an artificial intelligence (AI) based multilabel classification model is needed for automated diagnosis. To analyze the retinal malady, the system proposes a multiclass and multi-label arrangement method. Therefore, the classification frameworks based on features are explicitly described by ophthalmologists under the application of domain knowledge, which tends to be time-consuming, vulnerable generalization ability, and unfeasible in massive datasets. Therefore, the automated diagnosis of multi-retinal diseases becomes essential, which can be solved by the deep learning (DL) models. With this motivation, this paper presents an intelligent deep learning-based multi-retinal disease diagnosis (IDL-MRDD) framework using fundus images. The proposed model aims to classify the color fundus images into different classes namely AMD, DR, Glaucoma, Hypertensive Retinopathy, Normal, Others, and Pathological Myopia. Besides, the artificial flora algorithm with Shannon’s function (AFA-SF) based multi-level thresholding technique is employed for image segmentation and thereby the infected regions can be properly detected. In addition, SqueezeNet based feature extractor is employed to generate a collection of feature vectors. Finally, the stacked sparse Autoencoder (SSAE) model is applied as a classifier to distinguish the input images into distinct retinal diseases. The efficacy of the IDL-MRDD technique is carried out on a benchmark multi-retinal disease dataset, comprising data instances from different classes. The experimental values pointed out the superior outcome over the existing techniques with the maximum accuracy of 0.963.Keywords
Cite This Article
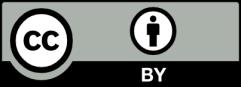
This work is licensed under a Creative Commons Attribution 4.0 International License , which permits unrestricted use, distribution, and reproduction in any medium, provided the original work is properly cited.