Open Access
ARTICLE
Ensemble of Handcrafted and Deep Learning Model for Histopathological Image Classification
1 Department of Computer Science Engineering, Vignan’s Nirula Institute of Technology and Science for Women, Guntur, 522005, India
2 Department of Computer Science Engineering, Gayatri Vidya Parishad College of Engineering for Women, Visakhapatnam, 530048, India
3 Department of Computer Science and Engineering, Ajay Binay Institute of Technology Cuttack, Odisha, 750314, India
4 Department of Computer Science and Engineering, Vignan’s Institute of Information Technology, Visakhapatnam, 530049, India
5 Energy Department, Technical College of Engineering, Duhok Polytechnic University, Duhok, Iraq
6 Computer Technology Engineering, College of Engineering Technology, Al-Kitab University, Iraq
7 Computer Technical Engineering Department, College of Technical Engineering, The Islamic University, Najaf, 54001, Iraq
8 College of Technical Engineering, The Islamic University, Najaf, Iraq
* Corresponding Author: Ahmed Alkhayyat. Email:
Computers, Materials & Continua 2022, 73(2), 4393-4406. https://doi.org/10.32604/cmc.2022.031109
Received 10 April 2022; Accepted 12 May 2022; Issue published 16 June 2022
Abstract
Histopathology is the investigation of tissues to identify the symptom of abnormality. The histopathological procedure comprises gathering samples of cells/tissues, setting them on the microscopic slides, and staining them. The investigation of the histopathological image is a problematic and laborious process that necessitates the expert’s knowledge. At the same time, deep learning (DL) techniques are able to derive features, extract data, and learn advanced abstract data representation. With this view, this paper presents an ensemble of handcrafted with deep learning enabled histopathological image classification (EHCDL-HIC) model. The proposed EHCDL-HIC technique initially performs Weiner filtering based noise removal technique. Once the images get smoothened, an ensemble of deep features and local binary pattern (LBP) features are extracted. For the classification process, the bidirectional gated recurrent unit (BGRU) model can be employed. At the final stage, the bacterial foraging optimization (BFO) algorithm is utilized for optimal hyperparameter tuning process which leads to improved classification performance, shows the novelty of the work. For validating the enhanced execution of the proposed EHCDL-HIC method, a set of simulations is performed. The experimentation outcomes highlighted the betterment of the EHCDL-HIC approach over the existing techniques with maximum accuracy of 94.78%. Therefore, the EHCDL-HIC model can be applied as an effective approach for histopathological image classification.Keywords
Cite This Article
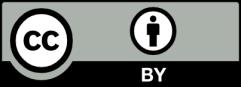