Open Access
ARTICLE
Compared Insights on Machine-Learning Anomaly Detection for Process Control Feature
1 School of Information, Liaoning University, Shenyang, 110036, China
2 School of Computer Science and Technology, Hainan University, Haikou, 570228, China
3 School of Physics, Liaoning University, Shenyang, 110036, China
4 Shenyang Institute of Automation Chinese Academy of Sciences, Shenyang, 110016, China
5 Department of Electrical Engineering and Information Technology, Karlsruhe Institute of Technology, Karlsruhe, 76131, Germany
* Corresponding Author: Jiangyuan Yao. Email:
Computers, Materials & Continua 2022, 73(2), 4033-4049. https://doi.org/10.32604/cmc.2022.030895
Received 05 April 2022; Accepted 09 May 2022; Issue published 16 June 2022
Abstract
Anomaly detection is becoming increasingly significant in industrial cyber security, and different machine-learning algorithms have been generally acknowledged as various effective intrusion detection engines to successfully identify cyber attacks. However, different machine-learning algorithms may exhibit their own detection effects even if they analyze the same feature samples. As a sequence, after developing one feature generation approach, the most effective and applicable detection engines should be desperately selected by comparing distinct properties of each machine-learning algorithm. Based on process control features generated by directed function transition diagrams, this paper introduces five different machine-learning algorithms as alternative detection engines to discuss their matching abilities. Furthermore, this paper not only describes some qualitative properties to compare their advantages and disadvantages, but also gives an in-depth and meticulous research on their detection accuracies and consuming time. In the verified experiments, two attack models and four different attack intensities are defined to facilitate all quantitative comparisons, and the impacts of detection accuracy caused by the feature parameter are also comparatively analyzed. All experimental results can clearly explain that SVM (Support Vector Machine) and WNN (Wavelet Neural Network) are suggested as two applicable detection engines under differing cases.Keywords
Cite This Article
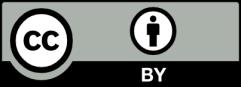
This work is licensed under a Creative Commons Attribution 4.0 International License , which permits unrestricted use, distribution, and reproduction in any medium, provided the original work is properly cited.