Open Access
ARTICLE
An Intelligent Tree Extractive Text Summarization Deep Learning
Department of Computer Sciences, College of Computer and Information Sciences, Princess Nourah Bint Abdulrahman University, P.O. Box 84428, Riyadh, 11671, Saudi Arabia
* Corresponding Author: Hanan Ahmed Hosni Mahmoud. Email:
Computers, Materials & Continua 2022, 73(2), 4231-4244. https://doi.org/10.32604/cmc.2022.030090
Received 18 March 2022; Accepted 17 May 2022; Issue published 16 June 2022
Abstract
In recent research, deep learning algorithms have presented effective representation learning models for natural languages. The deep learning-based models create better data representation than classical models. They are capable of automated extraction of distributed representation of texts. In this research, we introduce a new tree Extractive text summarization that is characterized by fitting the text structure representation in knowledge base training module, and also addresses memory issues that were not addresses before. The proposed model employs a tree structured mechanism to generate the phrase and text embedding. The proposed architecture mimics the tree configuration of the text-texts and provide better feature representation. It also incorporates an attention mechanism that offers an additional information source to conduct better summary extraction. The novel model addresses text summarization as a classification process, where the model calculates the probabilities of phrase and text-summary association. The model classification is divided into multiple features recognition such as information entropy, significance, redundancy and position. The model was assessed on two datasets, on the Multi-Doc Composition Query (MCQ) and Dual Attention Composition dataset (DAC) dataset. The experimental results prove that our proposed model has better summarization precision vs. other models by a considerable margin.Keywords
Cite This Article
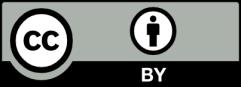
This work is licensed under a Creative Commons Attribution 4.0 International License , which permits unrestricted use, distribution, and reproduction in any medium, provided the original work is properly cited.