Open Access
ARTICLE
Methods and Means for Small Dynamic Objects Recognition and Tracking
Department of Computer Engineering, Lviv Polytechnic National University, Lviv, 79013, Ukraine
* Corresponding Author: Dmytro Kushnir. Email:
Computers, Materials & Continua 2022, 73(2), 3649-3665. https://doi.org/10.32604/cmc.2022.030016
Received 16 March 2022; Accepted 12 May 2022; Issue published 16 June 2022
Abstract
A literature analysis has shown that object search, recognition, and tracking systems are becoming increasingly popular. However, such systems do not achieve high practical results in analyzing small moving living objects ranging from 8 to 14 mm. This article examines methods and tools for recognizing and tracking the class of small moving objects, such as ants. To fulfill those aims, a customized You Only Look Once Ants Recognition (YOLO_AR) Convolutional Neural Network (CNN) has been trained to recognize Messor Structor ants in the laboratory using the LabelImg object marker tool. The proposed model is an extension of the You Only Look Once v4 (Yolov4) 512 × 512 model with an additional Self Regularized Non–Monotonic (Mish) activation function. Additionally, the scalable solution for continuous object recognizing and tracking was implemented. This solution is based on the OpenDatacam system, with extended Object Tracking modules that allow for tracking and counting objects that have crossed the custom boundary line. During the study, the methods of the alignment algorithm for finding the trajectory of moving objects were modified. I discovered that the Hungarian algorithm showed better results in tracking small objects than the K–D dimensional tree (k-d tree) matching algorithm used in OpenDataCam. Remarkably, such an algorithm showed better results with the implemented YOLO_AR model due to the lack of False Positives (FP). Therefore, I provided a new tracker module with a Hungarian matching algorithm verified on the Multiple Object Tracking (MOT) benchmark. Furthermore, additional customization parameters for object recognition and tracking results parsing and filtering were added, like boundary angle threshold (BAT) and past frames trajectory prediction (PFTP). Experimental tests confirmed the results of the study on a mobile device. During the experiment, parameters such as the quality of recognition and tracking of moving objects, the PFTP and BAT, and the configuration parameters of the neural network and boundary line model were analyzed. The results showed an increased tracking accuracy with the proposed methods by 50%. The study results confirmed the relevance of the topic and the effectiveness of the implemented methods and tools.Keywords
Cite This Article
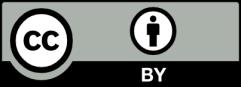
This work is licensed under a Creative Commons Attribution 4.0 International License , which permits unrestricted use, distribution, and reproduction in any medium, provided the original work is properly cited.