Open Access
ARTICLE
Adversarial Training Against Adversarial Attacks for Machine Learning-Based Intrusion Detection Systems
Department of Computer Science, Shaheed Zulfikar Ali Bhutto Institute of Science and Technology, Karachi, 75600, Pakistan
* Corresponding Author: Muhammad Shahzad Haroon. Email:
Computers, Materials & Continua 2022, 73(2), 3513-3527. https://doi.org/10.32604/cmc.2022.029858
Received 13 March 2022; Accepted 26 April 2022; Issue published 16 June 2022
Abstract
Intrusion detection system plays an important role in defending networks from security breaches. End-to-end machine learning-based intrusion detection systems are being used to achieve high detection accuracy. However, in case of adversarial attacks, that cause misclassification by introducing imperceptible perturbation on input samples, performance of machine learning-based intrusion detection systems is greatly affected. Though such problems have widely been discussed in image processing domain, very few studies have investigated network intrusion detection systems and proposed corresponding defence. In this paper, we attempt to fill this gap by using adversarial attacks on standard intrusion detection datasets and then using adversarial samples to train various machine learning algorithms (adversarial training) to test their defence performance. This is achieved by first creating adversarial sample based on Jacobian-based Saliency Map Attack (JSMA) and Fast Gradient Sign Attack (FGSM) using NSLKDD, UNSW-NB15 and CICIDS17 datasets. The study then trains and tests JSMA and FGSM based adversarial examples in seen (where model has been trained on adversarial samples) and unseen (where model is unaware of adversarial packets) attacks. The experiments includes multiple machine learning classifiers to evaluate their performance against adversarial attacks. The performance parameters include Accuracy, F1-Score and Area under the receiver operating characteristic curve (AUC) Score.Keywords
Cite This Article
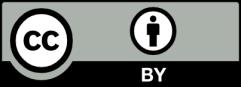
This work is licensed under a Creative Commons Attribution 4.0 International License , which permits unrestricted use, distribution, and reproduction in any medium, provided the original work is properly cited.