Open Access
ARTICLE
Cuckoo Optimized Convolution Support Vector Machine for Big Health Data Processing
1 Department of Computer Sciences, College of Computer and Information Sciences, Princess Nourah Bint Abdulrahman University, Riyadh, 11671, Saudi Arabia
2 Department of Industrial Engineering, College of Engineering at Alqunfudah, Umm Al-Qura University, Mecca, 24382, Saudi Arabia
3 Department of Information Systems, College of Science & Art at Mahayil, King Khalid University, Abha 62529, Saudi Arabia
4 Research Centre, Future University in Egypt, New Cairo, 11845, Egypt
5 Department of Computer and Self Development, Preparatory Year Deanship, Prince Sattam Bin Abdulaziz University, AlKharj, 16278, Saudi Arabia
6 Department of Information System, College of Computer Engineering and Sciences, Prince Sattam Bin Abdulaziz University, AlKharj, 16278, Saudi Arabia
* Corresponding Author: Manar Ahmed Hamza. Email:
Computers, Materials & Continua 2022, 73(2), 3039-3055. https://doi.org/10.32604/cmc.2022.029835
Received 12 March 2022; Accepted 22 April 2022; Issue published 16 June 2022
Abstract
Big health data collection and storing for further analysis is a challenging task because this knowledge is big and has many features. Several cloud-based IoT health providers have been described in the literature previously. Furthermore, there are a number of issues related to time consumed and overall network performance when it comes to big data information. In the existing method, less performed optimization algorithms were used for optimizing the data. In the proposed method, the Chaotic Cuckoo Optimization algorithm was used for feature selection, and Convolutional Support Vector Machine (CSVM) was used. The research presents a method for analyzing healthcare information that uses in future prediction. The major goal is to take a variety of data while improving efficiency and minimizing process time. The suggested method employs a hybrid method that is divided into two stages. In the first stage, it reduces the features by using the Chaotic Cuckoo Optimization algorithm with Levy flight, opposition-based learning, and distributor operator. In the second stage, CSVM is used which combines the benefits of convolutional neural network (CNN) and SVM. The CSVM modifies CNN’s convolution product to learn hidden deep inside data sources. For improved economic flexibility, greater protection, greater analytics with confidentiality, and lower operating cost, the suggested approach is built on fog computing. Overall results of the experiments show that the suggested method can minimize the number of features in the datasets, enhances the accuracy by 82%, and decrease the time of the process.Keywords
Cite This Article
E. Alabdulkreem, J. S. Alzahrani, M. M. Eltahir, A. Mohamed, M. Ahmed Hamza et al., "Cuckoo optimized convolution support vector machine for big health data processing," Computers, Materials & Continua, vol. 73, no.2, pp. 3039–3055, 2022. https://doi.org/10.32604/cmc.2022.029835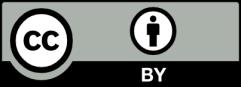