Open Access
ARTICLE
Manta Ray Foraging Optimization with Machine Learning Based Biomedical Data Classification
1 Department of Information Systems, College of Computer and Information Sciences, Princess Nourah bint Abdulrahman University, Riyadh, 11671, Saudi Arabia
2 Department of Industrial Engineering, College of Engineering at Alqunfudah, Umm Al-Qura University, Mecca, 24382, Saudi Arabia
3 Department of Information Systems, College of Science & Art at Mahayil, King Khalid University, Abha, 62529, Saudi Arabia
4 Research Centre, Future University in Egypt, New Cairo, 11845, Egypt
5 Department of Computer and Self Development, Preparatory Year Deanship, Prince Sattam bin Abdulaziz University, AlKharj, Saudi Arabia
6 Department of Information System, College of Computer Engineering and Sciences, Prince Sattam bin Abdulaziz University, AlKharj, Saudi Arabia
* Corresponding Author: Anwer Mustafa Hilal. Email:
Computers, Materials & Continua 2022, 73(2), 3275-3290. https://doi.org/10.32604/cmc.2022.029823
Received 12 March 2022; Accepted 19 April 2022; Issue published 16 June 2022
Abstract
The biomedical data classification process has received significant attention in recent times due to a massive increase in the generation of healthcare data from various sources. The developments of artificial intelligence (AI) and machine learning (ML) models assist in the effectual design of medical data classification models. Therefore, this article concentrates on the development of optimal Stacked Long Short Term Memory Sequence-to-Sequence Autoencoder (OSAE-LSTM) model for biomedical data classification. The presented OSAE-LSTM model intends to classify the biomedical data for the existence of diseases. Primarily, the OSAE-LSTM model involves min-max normalization based pre-processing to scale the data into uniform format. Followed by, the SAE-LSTM model is utilized for the detection and classification of diseases in biomedical data. At last, manta ray foraging optimization (MRFO) algorithm has been employed for hyperparameter optimization process. The utilization of MRFO algorithm assists in optimal selection of hypermeters involved in the SAE-LSTM model. The simulation analysis of the OSAE-LSTM model has been tested using a set of benchmark medical datasets and the results reported the improvements of the OSAE-LSTM model over the other approaches under several dimensions.Keywords
Cite This Article
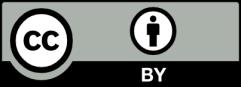
This work is licensed under a Creative Commons Attribution 4.0 International License , which permits unrestricted use, distribution, and reproduction in any medium, provided the original work is properly cited.