Open Access
ARTICLE
Aortic Dissection Diagnosis Based on Sequence Information and Deep Learning
1 Central South University of Forestry and Technology, Changsha, 410004, China
2 The Second Xiangya Hospital of Central South University, Changsha, 410011, China
3 Department of Mathematics and Computer Science, Northeastern State University, Tahlequah, 74464, USA
* Corresponding Author: Yun Tan. Email:
Computers, Materials & Continua 2022, 73(2), 2757-2771. https://doi.org/10.32604/cmc.2022.029727
Received 10 March 2022; Accepted 26 April 2022; Issue published 16 June 2022
Abstract
Aortic dissection (AD) is one of the most serious diseases with high mortality, and its diagnosis mainly depends on computed tomography (CT) results. Most existing automatic diagnosis methods of AD are only suitable for AD recognition, which usually require preselection of CT images and cannot be further classified to different types. In this work, we constructed a dataset of 105 cases with a total of 49021 slices, including 31043 slices expert-level annotation and proposed a two-stage AD diagnosis structure based on sequence information and deep learning. The proposed region of interest (RoI) extraction algorithm based on sequence information (RESI) can realize high-precision for RoI identification in the first stage. Then DenseNet-121 is applied for further diagnosis. Specially, the proposed method can judge the type of AD without preselection of CT images. The experimental results show that the accuracy of Stanford typing classification of AD is 89.19%, and the accuracy at the slice-level reaches 97.41%, which outperform the state-of-art methods. It can provide important decision-making information for the determination of further surgical treatment plan for patients.Keywords
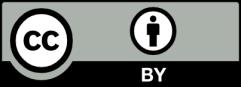