Open Access
ARTICLE
Sign Language Recognition and Classification Model to Enhance Quality of Disabled People
1 Department of Computer Sciences, College of Computer and Information Sciences, Princess Nourah Bint Abdulrahman University, Riyadh, 11671, Saudi Arabia
2 Department of Information Systems, College of Computing and Information System, Umm Al-Qura University, Saudi Arabia
3 Department of Computer Science, College of Science & Art at Mahayil, King Khalid University, Saudi Arabia
4 University of Tunis EL Manar, Higher Institute of Computer, Research Team on Intelligent Systems in Imaging and Artificial Vision (Siiva)–Lab Limtic, Aryanah, 2036, Tunisia
5 Department of Information Systems, College of Computer and Information Sciences, Princess Nourah Bint Abdulrahman University, Riyadh, 11671, Saudi Arabia
6 Research Centre, Future University in Egypt, New Cairo, 11745, Egypt
7 Department of Computer and Self Development, Preparatory Year Deanship, Prince Sattam bin Abdulaziz University, Alkharj, Saudi Arabia
* Corresponding Author: Anwer Mustafa Hilal. Email:
Computers, Materials & Continua 2022, 73(2), 3419-3432. https://doi.org/10.32604/cmc.2022.029438
Received 04 March 2022; Accepted 08 May 2022; Issue published 16 June 2022
Abstract
Sign language recognition can be considered as an effective solution for disabled people to communicate with others. It helps them in conveying the intended information using sign languages without any challenges. Recent advancements in computer vision and image processing techniques can be leveraged to detect and classify the signs used by disabled people in an effective manner. Metaheuristic optimization algorithms can be designed in a manner such that it fine tunes the hyper parameters, used in Deep Learning (DL) models as the latter considerably impacts the classification results. With this motivation, the current study designs the Optimal Deep Transfer Learning Driven Sign Language Recognition and Classification (ODTL-SLRC) model for disabled people. The aim of the proposed ODTL-SLRC technique is to recognize and classify sign languages used by disabled people. The proposed ODTL-SLRC technique derives EfficientNet model to generate a collection of useful feature vectors. In addition, the hyper parameters involved in EfficientNet model are fine-tuned with the help of HGSO algorithm. Moreover, Bidirectional Long Short Term Memory (BiLSTM) technique is employed for sign language classification. The proposed ODTL-SLRC technique was experimentally validated using benchmark dataset and the results were inspected under several measures. The comparative analysis results established the superior performance of the proposed ODTL-SLRC technique over recent approaches in terms of efficiency.Keywords
Cite This Article
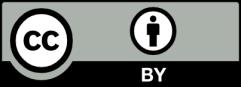