Open Access
ARTICLE
Binary Tomography Reconstruction with Limited-Data by a Convex Level-Set Method
1 Information and Systems, University of Tsukuba, Tsukuba, Ibaraki, 305-8573, Japan
2 Department of Mathematics, Faculty of Science, Sohag University, Sohag, 82524, Egypt
* Corresponding Author: Haytham A. Ali. Email:
Computers, Materials & Continua 2022, 73(2), 3741-3756. https://doi.org/10.32604/cmc.2022.029394
Received 03 March 2022; Accepted 20 April 2022; Issue published 16 June 2022
Abstract
This paper proposes a new level-set-based shape recovery approach that can be applied to a wide range of binary tomography reconstructions. In this technique, we derive generic evolution equations for shape reconstruction in terms of the underlying level-set parameters. We show that using the appropriate basis function to parameterize the level-set function results in an optimization problem with a small number of parameters, which overcomes many of the problems associated with the traditional level-set approach. More concretely, in this paper, we use Gaussian functions as a basis function placed at sparse grid points to represent the parametric level-set function and provide more flexibility in the binary representation of the reconstructed image. In addition, we suggest a convex optimization method that can overcome the problem of the local minimum of the cost function by successfully recovering the coefficients of the basis function. Finally, we illustrate the performance of the proposed method using synthetic images and real X-ray CT projection data. We show that the proposed reconstruction method compares favorably to various state-of-the-art reconstruction techniques for limited-data tomography, and it is also relatively stable in the presence of modest amounts of noise. Furthermore, the shape representation using a compact Gaussian radial basis function works well.Keywords
Cite This Article
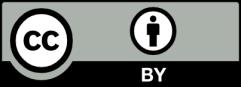