Open Access
ARTICLE
Criss-Cross Attentional Siamese Networks for Object Tracking
1 College of Computer Science and Information Technology, Central South University of Forestry & Technology, Changsha, 410004, China
2 Department of Mathematics and Computer Science, Northeastern State University, Tahlequah, 74464, OK, USA
* Corresponding Author: Jiaohua Qin. Email:
Computers, Materials & Continua 2022, 73(2), 2931-2946. https://doi.org/10.32604/cmc.2022.028896
Received 20 February 2022; Accepted 10 April 2022; Issue published 16 June 2022
Abstract
Visual object tracking is a hot topic in recent years. In the meanwhile, Siamese networks have attracted extensive attention in this field because of its balanced precision and speed. However, most of the Siamese network methods can only distinguish foreground from the non-semantic background. The fine-tuning and retraining of fully-convolutional Siamese networks for object tracking(SiamFC) can achieve higher precision under interferences, but the tracking accuracy is still not ideal, especially in the environment with more target interferences, dim light, and shadows. In this paper, we propose criss-cross attentional Siamese networks for object tracking (SiamCC). To solve the imbalance between foreground and non-semantic background, we use the feature enhancement module of criss-cross attention to greatly improve the accuracy of video object tracking in dim light and shadow environments. Experimental results show that the maximum running speed of SiamCC in the object tracking benchmark dataset is 90 frames/second. In terms of detection accuracy, the accuracy of shadow sequences is greatly improved, especially the accuracy score of sequence HUMAN8 is improved from 0.09 to 0.89 compared with the original SiamFC, and the success rate score is improved from 0.07 to 0.55.Keywords
Cite This Article
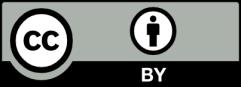