Open Access
ARTICLE
Optimal Deep Transfer Learning Model for Histopathological Breast Cancer Classification
1 Information Technology Department, Faculty of Computing and Information Technology, King Abdulaziz University, Jeddah 21589, Saudi Arabia
2 Center for Artificial Intelligence in Precision Medicines, King Abdulaziz University, Jeddah, 21589, Saudi Arabia
3 Mathematics Department, Faculty of Science, Al-Azhar University, Naser City, 11884, Cairo, Egypt
4 Biochemistry Department, Faculty of Science, King Abdulaziz University, Jeddah, 21589, Saudi Arabia
* Corresponding Author: Mahmoud Ragab. Email:
Computers, Materials & Continua 2022, 73(2), 2849-2864. https://doi.org/10.32604/cmc.2022.028855
Received 19 February 2022; Accepted 02 April 2022; Issue published 16 June 2022
Abstract
Earlier recognition of breast cancer is crucial to decrease the severity and optimize the survival rate. One of the commonly utilized imaging modalities for breast cancer is histopathological images. Since manual inspection of histopathological images is a challenging task, automated tools using deep learning (DL) and artificial intelligence (AI) approaches need to be designed. The latest advances of DL models help in accomplishing maximum image classification performance in several application areas. In this view, this study develops a Deep Transfer Learning with Rider Optimization Algorithm for Histopathological Classification of Breast Cancer (DTLRO-HCBC) technique. The proposed DTLRO-HCBC technique aims to categorize the existence of breast cancer using histopathological images. To accomplish this, the DTLRO-HCBC technique undergoes pre-processing and data augmentation to increase quantitative analysis. Then, optimal SqueezeNet model is employed for feature extractor and the hyperparameter tuning process is carried out using the Adadelta optimizer. Finally, rider optimization with deep feed forward neural network (RO-DFFNN) technique was utilized employed for breast cancer classification. The RO algorithm is applied for optimally adjusting the weight and bias values of the DFFNN technique. For demonstrating the greater performance of the DTLRO-HCBC approach, a sequence of simulations were carried out and the outcomes reported its promising performance over the current state of art approaches.Keywords
Cite This Article
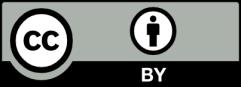
This work is licensed under a Creative Commons Attribution 4.0 International License , which permits unrestricted use, distribution, and reproduction in any medium, provided the original work is properly cited.