Open Access
ARTICLE
A Hybrid Particle Swarm Optimization to Forecast Implied Volatility Risk
1 University of Ha’il, Applied College, Hail City, Saudi Arabia
2 University of Tunis El Manar, Faculty of Economic Sciences and Management of Tunis, the International Finance Group, Tunis, Tunisia
3 Department of Computer and Network Engineering, College of Computer Science and Engineering, University of Jeddah, Jeddah, 21959, Saudi Arabia
4 Research Unit on Study of Systems and Renewable Energy, National College of Engineering of Monastir, University of Monastir, Monastir, Tunisia
5 LAREQUAD & FSEGT, University of Tunis El Manar, Tunis, Tunisia
6 University of Jendouba, Law Faculty, Management and Economic Sciences of Jendouba, Tunisia
* Corresponding Author: Kais Tissaoui. Email:
Computers, Materials & Continua 2022, 73(2), 4291-4309. https://doi.org/10.32604/cmc.2022.028830
Received 19 February 2022; Accepted 06 May 2022; Issue published 16 June 2022
Abstract
The application of optimization methods to prediction issues is a continually exploring field. In line with this, this paper investigates the connectedness between the infected cases of COVID-19 and US fear index from a forecasting perspective. The complex characteristics of implied volatility risk index such as non-linearity structure, time-varying and non-stationarity motivate us to apply a nonlinear polynomial Hammerstein model with known structure and unknown parameters. We use the Hybrid Particle Swarm Optimization (HPSO) tool to identify the model parameters of nonlinear polynomial Hammerstein model. Findings indicate that, following a nonlinear polynomial behaviour cascaded to an autoregressive with exogenous input (ARX) behaviour, the fear index in US financial market is significantly affected by COVID-19-infected cases in the US, COVID-19-infected cases in the world and COVID-19-infected cases in China, respectively. Statistical performance indicators provided by the developed models show that COVID-19-infected cases in the US are particularly powerful in predicting the Cboe volatility index compared to COVID-19-infected cases in the world and China (MAPE (2.1013%); R2 (91.78%) and RMSE (0.6363 percentage points)). The proposed approaches have also shown good convergence characteristics and accurate fits of the data.Keywords
Cite This Article
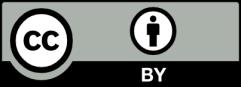
This work is licensed under a Creative Commons Attribution 4.0 International License , which permits unrestricted use, distribution, and reproduction in any medium, provided the original work is properly cited.