Open Access
ARTICLE
Deep Learning Enabled Object Detection and Tracking Model for Big Data Environment
1 Department of Computer Science and Engineering, Vignan’s Institute of Engineering for Women, Visakhapatnam, 530049, India
2 Department of Computer Science and Engineering, Vignan’s Institute of Information Technology, Visakhapatnam, 530049, India
3 Department of Computer Science and Information System, College of Applied Sciences, AlMaarefa University, Riyadh, 11597, Kingdom of Saudi Arabia
4 Department of Electronics and Communication Engineering, Kalasalingam Academy of Research and Education, Krishnankoil, 626126, Tamilnadu, India
5 Department of Computer Science, Christ University, Bangalore, 560029, India
6 Department of Entrepreneurship and Logistics, Plekhanov Russian University of Economics, 117997, Moscow, Russia
7 Department of Logistics, State University of Management, Moscow, 109542, Russia
* Corresponding Author: Irina V. Pustokhina. Email:
Computers, Materials & Continua 2022, 73(2), 2541-2554. https://doi.org/10.32604/cmc.2022.028570
Received 12 February 2022; Accepted 23 March 2022; Issue published 16 June 2022
Abstract
Recently, big data becomes evitable due to massive increase in the generation of data in real time application. Presently, object detection and tracking applications becomes popular among research communities and finds useful in different applications namely vehicle navigation, augmented reality, surveillance, etc. This paper introduces an effective deep learning based object tracker using Automated Image Annotation with Inception v2 based Faster RCNN (AIA-IFRCNN) model in big data environment. The AIA-IFRCNN model annotates the images by Discriminative Correlation Filter (DCF) with Channel and Spatial Reliability tracker (CSR), named DCF-CSRT model. The AIA-IFRCNN technique employs Faster RCNN for object detection and tracking, which comprises region proposal network (RPN) and Fast R-CNN. In addition, inception v2 model is applied as a shared convolution neural network (CNN) to generate the feature map. Lastly, softmax layer is applied to perform classification task. The effectiveness of the AIA-IFRCNN method undergoes experimentation against a benchmark dataset and the results are assessed under diverse aspects with maximum detection accuracy of 97.77%.Keywords
Cite This Article
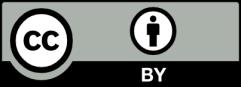
This work is licensed under a Creative Commons Attribution 4.0 International License , which permits unrestricted use, distribution, and reproduction in any medium, provided the original work is properly cited.