Open Access
ARTICLE
Optimal IoT Based Improved Deep Learning Model for Medical Image Classification
1 Department of Computer Science, King Khalid University, Saudi Arabia
2 School of Electrical Engineering, Vellore Institute of Technology, India
3 Department of Computer Science, Jazan University, Saudi Arabia
4 College of Computer Engineering and Science, Prince Sattam bin Abdulaziz University, Saudi Arabia
* Corresponding Author: Prasanalakshmi Balaji. Email:
Computers, Materials & Continua 2022, 73(2), 2275-2291. https://doi.org/10.32604/cmc.2022.028560
Received 12 February 2022; Accepted 28 March 2022; Issue published 16 June 2022
Abstract
Recently medical image classification plays a vital role in medical image retrieval and computer-aided diagnosis system. Despite deep learning has proved to be superior to previous approaches that depend on handcrafted features; it remains difficult to implement because of the high intra-class variance and inter-class similarity generated by the wide range of imaging modalities and clinical diseases. The Internet of Things (IoT) in healthcare systems is quickly becoming a viable alternative for delivering high-quality medical treatment in today’s e-healthcare systems. In recent years, the Internet of Things (IoT) has been identified as one of the most interesting research subjects in the field of health care, notably in the field of medical image processing. For medical picture analysis, researchers used a combination of machine and deep learning techniques as well as artificial intelligence. These newly discovered approaches are employed to determine diseases, which may aid medical specialists in disease diagnosis at an earlier stage, giving precise, reliable, efficient, and timely results, and lowering death rates. Based on this insight, a novel optimal IoT-based improved deep learning model named optimization-driven deep belief neural network (ODBNN) is proposed in this article. In context, primarily image quality enhancement procedures like noise removal and contrast normalization are employed. Then the pre-processed image is subjected to feature extraction techniques in which intensity histogram, an average pixel of RGB channels, first-order statistics, Grey Level Co-Occurrence Matrix, Discrete Wavelet Transform, and Local Binary Pattern measures are extracted. After extracting these sets of features, the May Fly optimization technique is adopted to select the most relevant features. The selected features are fed into the proposed classification algorithm in terms of classifying similar input images into similar classes. The proposed model is evaluated in terms of accuracy, precision, recall, and f-measure. The investigation evident the performance of incorporating optimization techniques for medical image classification is better than conventional techniques.Keywords
Cite This Article
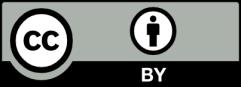
This work is licensed under a Creative Commons Attribution 4.0 International License , which permits unrestricted use, distribution, and reproduction in any medium, provided the original work is properly cited.