Open Access
ARTICLE
Fast CU Partition for VVC Using Texture Complexity Classification Convolutional Neural Network
1 The Information Department, Beijing University of Technology, Beijing, 100124, China
2 School of Physics and Electronic Information Engineering, Qinghai Minzu University, Xining, 810000, China
3 Advanced Information Network Beijing Laboratory, Beijing, 100124, China
4 Computational Intelligence and Intelligent Systems Beijing Key Laboratory, Beijing, 100124, China
5 Department of Computer Science, University of Pittsburgh, Pittsburgh, 15260, USA
* Corresponding Author: Pengyu Liu. Email:
Computers, Materials & Continua 2022, 73(2), 3545-3556. https://doi.org/10.32604/cmc.2022.028226
Received 05 February 2022; Accepted 18 March 2022; Issue published 16 June 2022
Abstract
Versatile video coding (H.266/VVC), which was newly released by the Joint Video Exploration Team (JVET), introduces quad-tree plus multi-type tree (QTMT) partition structure on the basis of quad-tree (QT) partition structure in High Efficiency Video Coding (H.265/HEVC). More complicated coding unit (CU) partitioning processes in H.266/VVC significantly improve video compression efficiency, but greatly increase the computational complexity compared. The ultra-high encoding complexity has obstructed its real-time applications. In order to solve this problem, a CU partition algorithm using convolutional neural network (CNN) is proposed in this paper to speed up the H.266/VVC CU partition process. Firstly, 64 × 64 CU is divided into smooth texture CU, mildly complex texture CU and complex texture CU according to the CU texture characteristics. Second, CU texture complexity classification convolutional neural network (CUTCC-CNN) is proposed to classify CUs. Finally, according to the classification results, the encoder is guided to skip different RDO search process. And optimal CU partition results will be determined. Experimental results show that the proposed method reduces the average coding time by 32.2% with only 0.55% BD-BR loss compared with VTM 10.2.Keywords
Cite This Article
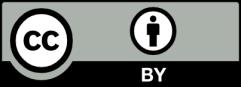
This work is licensed under a Creative Commons Attribution 4.0 International License , which permits unrestricted use, distribution, and reproduction in any medium, provided the original work is properly cited.