Open Access
ARTICLE
Non-Negative Minimum Volume Factorization (NMVF) for Hyperspectral Images (HSI) Unmixing: A Hybrid Approach
1 Department of Computer Science and Engineering, Chandigarh University, Punjab, 140413, India
2 Skill Faculty of Science and Technology, Shri Vishwakarma Skill University, Palwal, 121102, India
3 Department of Computer Science and Engineering, Soonchunhyang University, Asan, 31538, Korea
4 Department of ICT Convergence, Soonchunhyang University, Asan, 31538, Korea
5 Department of Mathematics, Faculty of Science, Mansoura University, Mansoura, 35516, Egypt
6 Department of Computational Mathematics, Science, and Engineering (CMSE), College of Engineering, Michigan State University, East Lansing, MI, 48824, USA
* Corresponding Author: Byeong-Gwon Kang. Email:
Computers, Materials & Continua 2022, 73(2), 3705-3720. https://doi.org/10.32604/cmc.2022.027936
Received 28 January 2022; Accepted 01 April 2022; Issue published 16 June 2022
Abstract
Spectral unmixing is essential for exploitation of remotely sensed data of Hyperspectral Images (HSI). It amounts to the identification of a position of spectral signatures that are pure and therefore called end members and their matching fractional, draft rules abundances for every pixel in HSI. This paper aims to unmix hyperspectral data using the minimal volume method of elementary scrutiny. Moreover, the problem of optimization is solved by the implementation of the sequence of small problems that are constrained quadratically. The hard constraint in the final step for the abundance fraction is then replaced with a loss function of hinge type that accounts for outliners and noise. Existing algorithms focus on estimating the endmembers (Ems) enumeration in a sight, discerning of spectral signs of EMs, besides assessment of fractional profusion for every EM in every pixel of a sight. Nevertheless, all the stages are performed by only a few algorithms in the process of hyperspectral unmixing. Therefore, the Non-negative Minimum Volume Factorization (NMVF) algorithm is further extended by fusing it with the nonnegative matrix of robust collaborative factorization that aims to perform all the three unmixing chain steps for hyperspectral images. The major contributions of this article are in this manner: (A) it performs Simplex analysis of minimum volume for hyperspectral images with unsupervised linear unmixing is employed. (B) The simplex analysis method is configured with an exaggerated form of the elementary which is delivered by vertical component analysis (VCA). (C) The inflating factor is chosen carefully inactivating the constraints in a large majority for relating to the source fractions abundance that speeds up the algorithm. (D) The final step is making simplex analysis method robust to outliners as well as noise that replaces the profusion element positivity hard restraint by a hinge kind soft restraint, preserving the local minima having good quality. (E) The matrix factorization method is applied that is capable of performing the three major phases of the hyperspectral separation sequence. The anticipated approach can find application in a scenario where the end members are known in advance, however, it assumes that the endmembers count is corresponding to an overestimated value. The proposed method is different from other conventional methods as it begins with the overestimation of the count of endmembers wherein removing the endmembers that are redundant by the means of collaborative regularization. As demonstrated by the experimental results, proposed approach yields competitive performance comparable with widely used methods.Keywords
Cite This Article
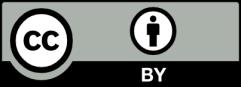
This work is licensed under a Creative Commons Attribution 4.0 International License , which permits unrestricted use, distribution, and reproduction in any medium, provided the original work is properly cited.