Open Access
ARTICLE
An Optimal Method for High-Resolution Population Geo-Spatial Data
Department of Disaster Management, Al-Balqa Applied University, Prince Al-Hussien Bin Abdullah II Academy for Civil Protection, Jordan
* Corresponding Author: Rami Sameer Ahmad Al Kloub. Email:
Computers, Materials & Continua 2022, 73(2), 2801-2820. https://doi.org/10.32604/cmc.2022.027847
Received 27 January 2022; Accepted 31 March 2022; Issue published 16 June 2022
Abstract
Mainland China has a poor distribution of meteorological stations. Existing models’ estimation accuracy for creating high-resolution surfaces of meteorological data is restricted for air temperature, and low for relative humidity and wind speed (few studies reported). This study compared the typical generalized additive model (GAM) and autoencoder-based residual neural network (hereafter, residual network for short) in terms of predicting three meteorological parameters, namely air temperature, relative humidity, and wind speed, using data from 824 monitoring stations across China’s mainland in 2015. The performance of the two models was assessed using a 10-fold cross-validation procedure. The air temperature models employ basic variables such as latitude, longitude, elevation, and the day of the year. The relative humidity models employ air temperature and ozone concentration as covariates, while the wind speed models use wind speed coarse-resolution reanalysis data as covariates, in addition to the fundamental variables. Spatial coordinates represent spatial variation, while the time index of the day captures time variation in our spatiotemporal models. In comparison to GAM, the residual network considerably improved prediction accuracy: on average, the coefficient of variation (CV) R2 of the three meteorological parameters rose by 0.21, CV root-mean square (RMSE) fell by 37%, and the relative humidity model improved the most. The accuracy of relative humidity models was considerably improved once the monthly index was included, demonstrating that varied amounts of temporal variables are crucial for relative humidity models. We also spoke about the benefits and drawbacks of using coarse resolution reanalysis data and closest neighbor values as variables. In comparison to classic GAMs, this study indicates that the residual network model may considerably increase the accuracy of national high spatial (1 km) and temporal (daily) resolution meteorological data. Our findings have implications for high-resolution and high-accuracy meteorological parameter mapping in China.Keywords
Cite This Article
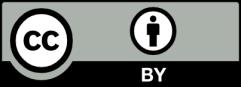
This work is licensed under a Creative Commons Attribution 4.0 International License , which permits unrestricted use, distribution, and reproduction in any medium, provided the original work is properly cited.