Open Access
ARTICLE
Automating Transfer Credit Assessment-A Natural Language Processing-Based Approach
Lakehead University, Thunder Bay, Ontario, P7B 5E1, Canada
* Corresponding Author: Dhivya Chandrasekaran. Email:
Computers, Materials & Continua 2022, 73(2), 2257-2274. https://doi.org/10.32604/cmc.2022.027236
Received 13 January 2022; Accepted 18 March 2022; Issue published 16 June 2022
Abstract
Student mobility or academic mobility involves students moving between institutions during their post-secondary education, and one of the challenging tasks in this process is to assess the transfer credits to be offered to the incoming student. In general, this process involves domain experts comparing the learning outcomes of the courses, to decide on offering transfer credits to the incoming students. This manual implementation is not only labor-intensive but also influenced by undue bias and administrative complexity. The proposed research article focuses on identifying a model that exploits the advancements in the field of Natural Language Processing (NLP) to effectively automate this process. Given the unique structure, domain specificity, and complexity of learning outcomes (LOs), a need for designing a tailor-made model arises. The proposed model uses a clustering-inspired methodology based on knowledge-based semantic similarity measures to assess the taxonomic similarity of LOs and a transformer-based semantic similarity model to assess the semantic similarity of the LOs. The similarity between LOs is further aggregated to form course to course similarity. Due to the lack of quality benchmark datasets, a new benchmark dataset containing seven course-to-course similarity measures is proposed. Understanding the inherent need for flexibility in the decision-making process the aggregation part of the model offers tunable parameters to accommodate different levels of leniency. While providing an efficient model to assess the similarity between courses with existing resources, this research work also steers future research attempts to apply NLP in the field of articulation in an ideal direction by highlighting the persisting research gaps.Keywords
Cite This Article
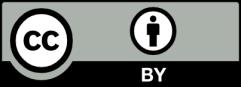
This work is licensed under a Creative Commons Attribution 4.0 International License , which permits unrestricted use, distribution, and reproduction in any medium, provided the original work is properly cited.