Open Access
ARTICLE
Pedestrian Physical Education Training Over Visualization Tool
1 Department of Humanities and Social Science, Al Ain University, Al Ain, 15551, UAE
2 Department of Computer Science, Air University, Islamabad, 44000, Pakistan
3 Department of Computer Science, College of Computer, Qassim University, Buraydah, 51452, Saudi Arabia
4 Department of Computer Science and Software Engineering, Al Ain University, Al Ain, 15551, UAE
5 Department of Computer Engineering, Korea Polytechnic University, Siheung-si, Gyeonggi-do, 237, Korea
* Corresponding Author: Jeongmin Park. Email:
Computers, Materials & Continua 2022, 73(2), 2389-2405. https://doi.org/10.32604/cmc.2022.027007
Received 08 January 2022; Accepted 14 March 2022; Issue published 16 June 2022
Abstract
E-learning approaches are one of the most important learning platforms for the learner through electronic equipment. Such study techniques are useful for other groups of learners such as the crowd, pedestrian, sports, transports, communication, emergency services, management systems and education sectors. E-learning is still a challenging domain for researchers and developers to find new trends and advanced tools and methods. Many of them are currently working on this domain to fulfill the requirements of industry and the environment. In this paper, we proposed a method for pedestrian behavior mining of aerial data, using deep flow feature, graph mining technique, and convocational neural network. For input data, the state-of-the-art crowd activity University of Minnesota (UMN) dataset is adopted, which contains the aerial indoor and outdoor view of the pedestrian, for simplification of extra information and computational cost reduction the pre-processing is applied. Deep flow features are extracted to find more accurate information. Furthermore, to deal with repetition in features data and features mining the graph mining algorithm is applied, while Convolution Neural Network (CNN) is applied for pedestrian behavior mining. The proposed method shows 84.50% of mean accuracy and a 15.50% of error rate. Therefore, the achieved results show more accuracy as compared to state-of-the-art classification algorithms such as decision tree, artificial neural network (ANN).Keywords
Cite This Article
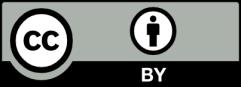
This work is licensed under a Creative Commons Attribution 4.0 International License , which permits unrestricted use, distribution, and reproduction in any medium, provided the original work is properly cited.