Open Access
ARTICLE
Segmentation of Remote Sensing Images Based on U-Net Multi-Task Learning
1 College of Information Technology, Jilin Agricultural University, Changchun, 130118, China
2 Jilin Province Agricultural Internet of Things Technology Collaborative Innovation Center, Changchun, 130118, China
3 Jilin Province Intelligent Environmental Engineering Research Center, Changchun, 130118, China
4 Jilin Province Information Technology and Intelligent Agriculture Engineering Research Center, Changchun, 130118, China
5 College of Information Technology, Wuzhou University, Wuzhou, 543003, China
6 Guangxi Key Laboratory of Machine Vision and Intelligent Control, Wuzhou, 543003, China
7 Department of Agricultural Economics and Animal Production, University of Limpopo, Sovenga, Polokwane, 0727, South Africa
* Corresponding Author: Mu Ye. Email:
Computers, Materials & Continua 2022, 73(2), 3263-3274. https://doi.org/10.32604/cmc.2022.026881
Received 06 January 2022; Accepted 23 February 2022; Issue published 16 June 2022
Abstract
In order to accurately segment architectural features in high-resolution remote sensing images, a semantic segmentation method based on U-net network multi-task learning is proposed. First, a boundary distance map was generated based on the remote sensing image of the ground truth map of the building. The remote sensing image and its truth map were used as the input in the U-net network, followed by the addition of the building ground prediction layer at the end of the U-net network. Based on the ResNet network, a multi-task network with the boundary distance prediction layer was built. Experiments involving the ISPRS aerial remote sensing image building and feature annotation data set show that compared with the full convolutional network combined with the multi-layer perceptron method, the intersection ratio of VGG16 network, VGG16 + boundary prediction, ResNet50 and the method in this paper were increased by 5.15%, 6.946%, 6.41% and 7.86%. The accuracy of the networks was increased to 94.71%, 95.39%, 95.30% and 96.10% respectively, which resulted in high-precision extraction of building features.Keywords
Cite This Article
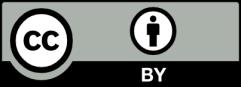