Open Access
ARTICLE
Threefold Optimized Forecasting of Electricity Consumption in Higher Education Institutions
1 Faculty of Electrical and Computer Engineering, NED University of Engineering and Technology, Karachi, 75270, Pakistan
2 Neurocomputation Lab, National Center of Artificial Intelligence, Karachi, 75270, Pakistan
* Corresponding Author: Majida Kazmi. Email:
Computers, Materials & Continua 2022, 73(2), 2351-2370. https://doi.org/10.32604/cmc.2022.026265
Received 20 December 2021; Accepted 30 March 2022; Issue published 16 June 2022
Abstract
Energy management benefits both consumers and utility companies alike. Utility companies remain interested in identifying and reducing energy waste and theft, whereas consumers’ interest remain in lowering their energy expenses. A large supply-demand gap of over 6 GW exists in Pakistan as reported in 2018. Reducing this gap from the supply side is an expensive and complex task. However, efficient energy management and distribution on demand side has potential to reduce this gap economically. Electricity load forecasting models are increasingly used by energy managers in taking real-time tactical decisions to ensure efficient use of resources. Advancement in Machine-learning (ML) technology has enabled accurate forecasting of electricity consumption. However, the impact of computation cost afforded by these ML models is often ignored in favour of accuracy. This study considers both accuracy and computation cost as concurrently significant factors because together they shape the technology environment as well as create economic impact. Thus, a three-fold optimized load forecasting model is proposed which includes (1) application specific parameters selection, (2) impact of different dataset granularities and (3) implementation of specific data preparation. It deploys and compares the widely used back-propagation Artificial Neural Network (ANN) and Random Forest (RF) models for the prediction of electricity consumption of buildings within a university. In addition to the temporal and historical power consumption date as input parameters, the study also embeds weather data as well as university operational calendars resulting in improved performance. The outcomes are indicative that the granularity i.e. the scale of details in data, and set of reduced and full input parameters impact performance accuracies differently for ANN and RF models. Experimental results show that overall RF model performed better both in terms of accuracy as well as computational time for a 1-min, 15-min and 1-h dataset granularities with the mean absolute percentage error (MAPE) of 2.42, 3.70 and 4.62 in 11.1 s, 1.14 s and 0.3 s respectively, thus well suited for a real-time energy monitoring application.Keywords
Cite This Article
M. Kazmi, H. Raza Khan, L. , M. Hashir Bin Khalid and S. Ahmed Qazi, "Threefold optimized forecasting of electricity consumption in higher education institutions," Computers, Materials & Continua, vol. 73, no.2, pp. 2351–2370, 2022. https://doi.org/10.32604/cmc.2022.026265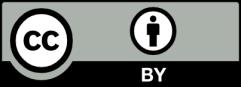