Open Access
ARTICLE
Meta-heuristics for Feature Selection and Classification in Diagnostic Breast Cancer
1 Department of Computer Sciences, College of Computer and Information Sciences, Princess Nourah Bint Abdulrahman University, Riyadh, 11671, Saudi Arabia
2 Department of Communications and Electronics, Delta Higher Institute of Engineering and Technology, Mansoura, 35111, Egypt
3 Faculty of Artificial Intelligence, Delta University for Science and Technology, Mansoura, 35712, Egypt
4 Communications and Computers Engineering Department, Faculty of Engineering, Delta University for Science and Technology, International Coastal Road, Gamasa City, Dakhliya, Egypt
5 Faculty of Applied Health Science Technology, Delta University for Science and Technology, Mansoura, 35712, Egypt
6 Faculty of Medicine, Head of Internal Medicine Department, Delta University for Science and Technology, Mansoura, Egypt
7 Computer Engineering and Control Systems Department, Faculty of Engineering, Mansoura University, 35516, Mansoura, Egypt
8 Department of Computer Science, Faculty of Computer and Information Sciences, Ain Shams University, 11566, Cairo, Egypt
9 Department of Computer Science, College of Computing and Information Technology, Shaqra University, 11961, Saudi Arabia
* Corresponding Author: Amel Ali Alhussan. Email:
Computers, Materials & Continua 2022, 73(1), 749-765. https://doi.org/10.32604/cmc.2022.029605
Received 07 March 2022; Accepted 07 April 2022; Issue published 18 May 2022
Abstract
One of the most common kinds of cancer is breast cancer. The early detection of it may help lower its overall rates of mortality. In this paper, we robustly propose a novel approach for detecting and classifying breast cancer regions in thermal images. The proposed approach starts with data preprocessing the input images and segmenting the significant regions of interest. In addition, to properly train the machine learning models, data augmentation is applied to increase the number of segmented regions using various scaling ratios. On the other hand, to extract the relevant features from the breast cancer cases, a set of deep neural networks (VGGNet, ResNet-50, AlexNet, and GoogLeNet) are employed. The resulting set of features is processed using the binary dipper throated algorithm to select the most effective features that can realize high classification accuracy. The selected features are used to train a neural network to finally classify the thermal images of breast cancer. To achieve accurate classification, the parameters of the employed neural network are optimized using the continuous dipper throated optimization algorithm. Experimental results show the effectiveness of the proposed approach in classifying the breast cancer cases when compared to other recent approaches in the literature. Moreover, several experiments were conducted to compare the performance of the proposed approach with the other approaches. The results of these experiments emphasized the superiority of the proposed approach.Keywords
Cite This Article
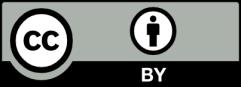
This work is licensed under a Creative Commons Attribution 4.0 International License , which permits unrestricted use, distribution, and reproduction in any medium, provided the original work is properly cited.