Open Access
ARTICLE
Optimal Fusion-Based Handcrafted with Deep Features for Brain Cancer Classification
1 Information Technology Department, Faculty of Computing and Information Technology, King Abdulaziz University, Jeddah, 21589, Saudi Arabia
2 Center for Artificial Intelligence in Precision Medicines, King Abdulaziz University, Jeddah, 21589, Saudi Arabia
3 Mathematics Department, Faculty of Science, Al-Azhar University, Naser City, 11884, Cairo, Egypt
4 Computer Science Department, Faculty of Computing and Information Technology, King Abdulaziz University, Jeddah, 21589, Saudi Arabia
5 Biochemistry Department, Faculty of Science, King Abdulaziz University, Jeddah, 21589, Saudi Arabia
6 Department of Mathematics, College of Science and Arts in Ar Rass, Qassim University, Buryadah, 52571, Saudi Arabia
* Corresponding Author: Mahmoud Ragab. Email:
Computers, Materials & Continua 2022, 73(1), 801-815. https://doi.org/10.32604/cmc.2022.029140
Received 25 February 2022; Accepted 06 April 2022; Issue published 18 May 2022
Abstract
Brain cancer detection and classification is done utilizing distinct medical imaging modalities like computed tomography (CT), or magnetic resonance imaging (MRI). An automated brain cancer classification using computer aided diagnosis (CAD) models can be designed to assist radiologists. With the recent advancement in computer vision (CV) and deep learning (DL) models, it is possible to automatically detect the tumor from images using a computer-aided design. This study focuses on the design of automated Henry Gas Solubility Optimization with Fusion of Handcrafted and Deep Features (HGSO-FHDF) technique for brain cancer classification. The proposed HGSO-FHDF technique aims for detecting and classifying different stages of brain tumors. The proposed HGSO-FHDF technique involves Gabor filtering (GF) technique for removing the noise and enhancing the quality of MRI images. In addition, Tsallis entropy based image segmentation approach is applied to determine injured brain regions in the MRI image. Moreover, a fusion of handcrafted with deep features using Residual Network (ResNet) is utilized as feature extractors. Finally, HGSO algorithm with kernel extreme learning machine (KELM) model was utilized for identifying the presence of brain tumors. For examining the enhanced brain tumor classification performance, a comprehensive set of simulations take place on the BRATS 2015 dataset.Keywords
Cite This Article
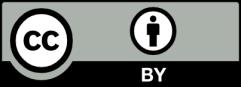