Open Access
ARTICLE
Automated Machine Learning for Epileptic Seizure Detection Based on EEG Signals
1 University of Science and Technology Beijing, Beijing, 100083, China
2 Hwa Create Co., Ltd, Beijing, 100193, China
3 Amphenol Global Interconnect Systems, San Jose, CA 95131, US
* Corresponding Author: Xiang Wang. Email:
Computers, Materials & Continua 2022, 73(1), 1995-2011. https://doi.org/10.32604/cmc.2022.029073
Received 23 February 2022; Accepted 31 March 2022; Issue published 18 May 2022
Abstract
Epilepsy is a common neurological disease and severely affects the daily life of patients. The automatic detection and diagnosis system of epilepsy based on electroencephalogram (EEG) is of great significance to help patients with epilepsy return to normal life. With the development of deep learning technology and the increase in the amount of EEG data, the performance of deep learning based automatic detection algorithm for epilepsy EEG has gradually surpassed the traditional hand-crafted approaches. However, the neural architecture design for epilepsy EEG analysis is time-consuming and laborious, and the designed structure is difficult to adapt to the changing EEG collection environment, which limits the application of the epilepsy EEG automatic detection system. In this paper, we explore the possibility of Automated Machine Learning (AutoML) playing a role in the task of epilepsy EEG detection. We apply the neural architecture search (NAS) algorithm in the AutoKeras platform to design the model for epilepsy EEG analysis and utilize feature interpretability methods to ensure the reliability of the searched model. The experimental results show that the model obtained through NAS outperforms the baseline model in performance. The searched model improves classification accuracy, F1-score and Cohen’s kappa coefficient by 7.68%, 7.82% and 9.60% respectively than the baseline model. Furthermore, NAS-based model is capable of extracting EEG features related to seizures for classification.Keywords
Cite This Article
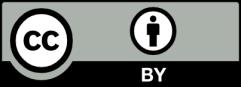
This work is licensed under a Creative Commons Attribution 4.0 International License , which permits unrestricted use, distribution, and reproduction in any medium, provided the original work is properly cited.