Open Access
ARTICLE
Single and Mitochondrial Gene Inheritance Disorder Prediction Using Machine Learning
1 Riphah School of Computing & Innovation, Faculty of Computing, Riphah International University Lahore Campus, Lahore, 54000, Pakistan
2 Pattern Recognition and Machine Learning Lab, Department of Software, Gachon University, Seongnam, 13120, Gyeonggido, Korea
3 Faculty of Computing, Riphah International University, Islamabad, 45000, Pakistan
4 School of Information Technology, Skyline University College, Sharjah, 1797, UAE
5 Network and Communication Technology Lab, Center for Cyber Security, Faculty of Information Science and Technology Universiti Kebangsaan Malaysia, 43600, Malaysia
6 Canadian University, Dubai, 00000, UAE
7 Cyber-Physical Systems, Khalifa University, Abu Dhabi, 127788, UAE
* Corresponding Author: Hussam Al Hamadi. Email:
Computers, Materials & Continua 2022, 73(1), 953-963. https://doi.org/10.32604/cmc.2022.028958
Received 22 February 2022; Accepted 24 March 2022; Issue published 18 May 2022
Abstract
One of the most difficult jobs in the post-genomic age is identifying a genetic disease from a massive amount of genetic data. Furthermore, the complicated genetic disease has a very diverse genotype, making it challenging to find genetic markers. This is a challenging process since it must be completed effectively and efficiently. This research article focuses largely on which patients are more likely to have a genetic disorder based on numerous medical parameters. Using the patient’s medical history, we used a genetic disease prediction algorithm that predicts if the patient is likely to be diagnosed with a genetic disorder. To predict and categorize the patient with a genetic disease, we utilize several deep and machine learning techniques such as Artificial neural network (ANN), K-nearest neighbors (KNN), and Support vector machine (SVM). To enhance the accuracy of predicting the genetic disease in any patient, a highly efficient approach was utilized to control how the model can be used. To predict genetic disease, deep and machine learning approaches are performed. The most productive tool model provides more precise efficiency. The simulation results demonstrate that by using the proposed model with the ANN, we achieve the highest model performance of 85.7%, 84.9%, 84.3% accuracy of training, testing and validation respectively. This approach will undoubtedly transform genetic disorder prediction and give a real competitive strategy to save patients’ lives.Keywords
Cite This Article
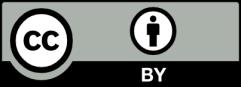
This work is licensed under a Creative Commons Attribution 4.0 International License , which permits unrestricted use, distribution, and reproduction in any medium, provided the original work is properly cited.