Open Access
ARTICLE
Improved Prediction of Metamaterial Antenna Bandwidth Using Adaptive Optimization of LSTM
1 Department of Computer Sciences, College of Computer and Information Sciences, Princess Nourah Bint Abdulrahman University, Riyadh, 11671, Saudi Arabia
2 Department of Communications and Electronics, Delta Higher Institute of Engineering and Technology, Mansoura, 35111, Egypt
3 Faculty of Artificial Intelligence, Delta University for Science and Technology, Mansoura, 35712, Egypt
4 Computer Engineering and Control Systems Department, Faculty of Engineering, Mansoura University, Mansoura, 35516, Egypt
5 Department of Computer Systems Engineering, Faculty of Engineering at Shoubra, Benha University, Egypt
6 Department of Computer Science, Faculty of Computer and Information Sciences, Ain Shams University, Cairo,11566, Egypt
7 Department of Computer Science, College of Computing and Information Technology, Shaqra University, 11961,Saudi Arabia
* Corresponding Author: Amel Ali Alhussan. Email:
Computers, Materials & Continua 2022, 73(1), 865-881. https://doi.org/10.32604/cmc.2022.028550
Received 12 February 2022; Accepted 14 March 2022; Issue published 18 May 2022
Abstract
The design of an antenna requires a careful selection of its parameters to retain the desired performance. However, this task is time-consuming when the traditional approaches are employed, which represents a significant challenge. On the other hand, machine learning presents an effective solution to this challenge through a set of regression models that can robustly assist antenna designers to find out the best set of design parameters to achieve the intended performance. In this paper, we propose a novel approach for accurately predicting the bandwidth of metamaterial antenna. The proposed approach is based on employing the recently emerged guided whale optimization algorithm using adaptive particle swarm optimization to optimize the parameters of the long-short-term memory (LSTM) deep network. This optimized network is used to retrieve the metamaterial bandwidth given a set of features. In addition, the superiority of the proposed approach is examined in terms of a comparison with the traditional multilayer perceptron (ML), K-nearest neighbors (K-NN), and the basic LSTM in terms of several evaluation criteria such as root mean square error (RMSE), mean absolute error (MAE), and mean bias error (MBE). Experimental results show that the proposed approach could achieve RMSE of (0.003018), MAE of (0.001871), and MBE of (0.000205). These values are better than those of the other competing models.Keywords
Cite This Article
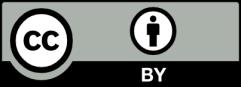
This work is licensed under a Creative Commons Attribution 4.0 International License , which permits unrestricted use, distribution, and reproduction in any medium, provided the original work is properly cited.