Open Access
ARTICLE
Novel Computing for the Delay Differential Two-Prey and One-Predator System
1 Department of Statistics, Faculty of Science, Khon Kaen University, Khon Kaen, 40002, Thailand
2 Department of Mathematics and Statistics, Hazara University, Mansehra, Pakistan
3 Future Technology Research Center, National Yunlin University of Science and Technology, Douliou, 64002, Taiwan
4 Faculty of Engineering and Natural Sciences, Bahcesehir University, Istanbul, Turkey
5 Department of Mathematics, Faculty of Science, Khon Kaen University, Khon Kaen, 40002, Thailand
* Corresponding Author: Thongchai Botmart. Email:
Computers, Materials & Continua 2022, 73(1), 249-263. https://doi.org/10.32604/cmc.2022.028513
Received 11 February 2022; Accepted 24 March 2022; Issue published 18 May 2022
Abstract
The aim of these investigations is to find the numerical performances of the delay differential two-prey and one-predator system. The delay differential models are very significant and always difficult to solve the dynamical kind of ecological nonlinear two-prey and one-predator system. Therefore, a stochastic numerical paradigm based artificial neural network (ANN) along with the Levenberg-Marquardt backpropagation (L-MB) neural networks (NNs), i.e., L-MBNNs is proposed to solve the dynamical two-prey and one-predator model. Three different cases based on the dynamical two-prey and one-predator system have been discussed to check the correctness of the L-MBNNs. The statistic measures of these outcomes of the dynamical two-prey and one-predator model are chosen as 13% for testing, 12% for authorization and 75% for training. The exactness of the proposed results of L-MBNNs approach for solving the dynamical two-prey and one-predator model is observed with the comparison of the Runge-Kutta method with absolute error ranges between 10−05 to 10−07. To check the validation, constancy, validity, exactness, competence of the L-MBNNs, the obtained state transitions (STs), regression actions, correlation presentations, MSE and error histograms (EHs) are also provided.Keywords
Cite This Article
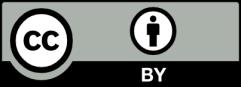
This work is licensed under a Creative Commons Attribution 4.0 International License , which permits unrestricted use, distribution, and reproduction in any medium, provided the original work is properly cited.