Open Access
ARTICLE
Bird Swarm Algorithm with Fuzzy Min-Max Neural Network for Financial Crisis Prediction
1 Department of Computing Technologies, Associate Professor, SRM Institute of Science and Technology, Kattankulathur, 603203, India
2 Department of Information Technology, Kalasalingam Academy of Research and Education, 626126, India
3 Department of Computer Science and Engineering, Sphoorthy Engineering College, Hyderabad, Telangana, 501510, India
4 Department of Electrical and Electronics Engineering, J B Institute of Engineering and Technology, Hyderabad, Telangana, 500075, India
5 Department of Computer Science and Information System, College of Applied Sciences, AlMaarefa University, Riyadh, 11597, Kingdom of Saudi Arabia
6 Department of Entrepreneurship and Logistics, Plekhanov Russian University of Economics, 117997, Moscow, Russia
7 Department of Logistics, State University of Management, 109542, Moscow, Russia
* Corresponding Author: Irina V. Pustokhina. Email:
Computers, Materials & Continua 2022, 73(1), 1541-1555. https://doi.org/10.32604/cmc.2022.028338
Received 08 February 2022; Accepted 10 March 2022; Issue published 18 May 2022
Abstract
Financial crisis prediction (FCP) models are used for predicting or forecasting the financial status of a company or financial firm. It is considered a challenging issue in the financial sector. Statistical and machine learning (ML) models can be employed for the design of accurate FCP models. Though numerous works have existed in the literature, it is needed to design effective FCP models adaptable to different datasets. This study designs a new bird swarm algorithm (BSA) with fuzzy min-max neural network (FMM-NN) model, named BSA-FMMNN for FCP. The major intention of the BSA-FMMNN model is to determine the financial status of a firm or company. The presented BSA-FMMNN model primarily undergoes min-max normalization to transform the data into uniformity range. Besides, k-medoid clustering approach is employed for the outlier removal process. Finally, the classification process is carried out using the FMMNN model, and the parameters involved in it are tuned by the use of BSA. The utilization of proficient parameter selection process using BSA demonstrate the novelty of the study. The experimental result analysis of the BSA-FMMNN model is validated using benchmark dataset and the comparative outcomes highlighted the supremacy of the BSA-FMMNN model over the recent approaches.Keywords
Cite This Article
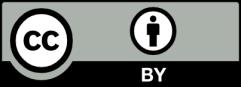