Open Access
ARTICLE
Energy Price Forecasting Through Novel Fuzzy Type-1 Membership Functions
1 Centre of Research in Data Science, Computer, and Information Sciences Department, Universiti Teknologi PETRONAS, Seri Iskandar, 32610, Perak, Malaysia
2 Petroleum Engineering Department, Universiti Teknologi PETRONAS, Seri Iskandar, 32610, Perak, Malaysia
3 Institute of Computing, Kohat University of Science and Technology, Kohat, 26000, K.P.K, Pakistan
* Corresponding Author: Mohd Hilmi Hasan. Email:
Computers, Materials & Continua 2022, 73(1), 1799-1815. https://doi.org/10.32604/cmc.2022.028292
Received 07 February 2022; Accepted 10 March 2022; Issue published 18 May 2022
Abstract
Electricity price forecasting is a subset of energy and power forecasting that focuses on projecting commercial electricity market present and future prices. Electricity price forecasting have been a critical input to energy corporations’ strategic decision-making systems over the last 15 years. Many strategies have been utilized for price forecasting in the past, however Artificial Intelligence Techniques (Fuzzy Logic and ANN) have proven to be more efficient than traditional techniques (Regression and Time Series). Fuzzy logic is an approach that uses membership functions (MF) and fuzzy inference model to forecast future electricity prices. Fuzzy c-means (FCM) is one of the popular clustering approach for generating fuzzy membership functions. However, the fuzzy c-means algorithm is limited to producing only one type of MFs, Gaussian MF. The generation of various fuzzy membership functions is critical since it allows for more efficient and optimal problem solutions. As a result, for the best and most improved results for electricity price forecasting, an approach to generate multiple type-1 fuzzy MFs using FCM algorithm is required. Therefore, the objective of this paper is to propose an approach for generating type-1 fuzzy triangular and trapezoidal MFs using FCM algorithm to overcome the limitations of the FCM algorithm. The approach is used to compute and improve forecasting accuracy for electricity prices, where Australian Energy Market Operator (AEMO) data is used. The results show that the proposed approach of using FCM to generate type-1 fuzzy MFs is effective and can be adopted.Keywords
Cite This Article
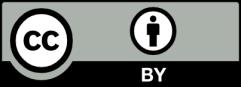
This work is licensed under a Creative Commons Attribution 4.0 International License , which permits unrestricted use, distribution, and reproduction in any medium, provided the original work is properly cited.