Open Access
ARTICLE
Rough Sets Hybridization with Mayfly Optimization for Dimensionality Reduction
1 Faculty of Computers and Artificial Intelligence, Benha University, Benha, 13518, Egypt
2 College of Computer and Information Sciences, Prince Sultan University, Riyadh, 11586, Saudi Arabia
3 Faculty of Computer Studies, Arab Open University, Cairo, Egypt
4 Faculty of Information Technology and Computer Science, Nile University, Shikh Zaid, Egypt
* Corresponding Authors: Ahmad Taher Azar. Email: ,
Computers, Materials & Continua 2022, 73(1), 1087-1108. https://doi.org/10.32604/cmc.2022.028184
Received 04 February 2022; Accepted 24 March 2022; Issue published 18 May 2022
Abstract
Big data is a vast amount of structured and unstructured data that must be dealt with on a regular basis. Dimensionality reduction is the process of converting a huge set of data into data with tiny dimensions so that equal information may be expressed easily. These tactics are frequently utilized to improve classification or regression challenges while dealing with machine learning issues. To achieve dimensionality reduction for huge data sets, this paper offers a hybrid particle swarm optimization-rough set PSO-RS and Mayfly algorithm-rough set MA-RS. A novel hybrid strategy based on the Mayfly algorithm (MA) and the rough set (RS) is proposed in particular. The performance of the novel hybrid algorithm MA-RS is evaluated by solving six different data sets from the literature. The simulation results and comparison with common reduction methods demonstrate the proposed MA-RS algorithm’s capacity to handle a wide range of data sets. Finally, the rough set approach, as well as the hybrid optimization techniques PSO-RS and MA-RS, were applied to deal with the massive data problem. MA-hybrid RS’s method beats other classic dimensionality reduction techniques, according to the experimental results and statistical testing studies.Keywords
Cite This Article
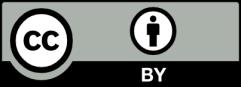