Open Access
ARTICLE
Improved Harmony Search with Optimal Deep Learning Enabled Classification Model
1 Information Technology Department, Faculty of Computing and Information Technology, King Abdulaziz University, Jeddah, 21589, Saudi Arabia
2 Centre for Artificial Intelligence in Precision Medicines, King Abdulaziz University, Jeddah, 21589, Saudi Arabia
3 Mathematics Department, Faculty of Science, Al-Azhar University, Naser City, 11884, Cairo, Egypt
4 Information Systems Department, Faculty of Computing and Information Technology, King Abdulaziz University, Jeddah, 21589, Saudi Arabia
* Corresponding Author: Mahmoud Ragab. Email:
Computers, Materials & Continua 2022, 73(1), 1783-1797. https://doi.org/10.32604/cmc.2022.028055
Received 01 February 2022; Accepted 08 March 2022; Issue published 18 May 2022
Abstract
Due to drastic increase in the generation of data, it is tedious to examine and derive high level knowledge from the data. The rising trends of high dimension data gathering and problem representation necessitates feature selection process in several machine learning processes. The feature selection procedure establishes a generally encountered issue of global combinatorial optimization. The FS process can lessen the number of features by the removal of unwanted and repetitive data. In this aspect, this article introduces an improved harmony search based global optimization for feature selection with optimal deep learning (IHSFS-ODL) enabled classification model. The proposed IHSFS-ODL technique intends to reduce the curse of dimensionality and enhance classification outcomes. In addition, the IHSFS-ODL technique derives an IHSFS technique by the use of local search method with traditional harmony search algorithm (HSA) for global optimization. Besides, ODL based classifier including quantum behaved particle swarm optimization (QPSO) with gated recurrent unit (GRU) is applied for data classification process. The utilization of HSA for the choice of features and QPSO algorithm for hyper parameter tuning processes helps to accomplish maximum classification performance. In order to demonstrate the enhanced outcomes of the IHSFS-ODL technique, a series of simulations were carried out and the results reported the betterment over its recent state of art approaches.Keywords
Cite This Article
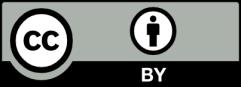
This work is licensed under a Creative Commons Attribution 4.0 International License , which permits unrestricted use, distribution, and reproduction in any medium, provided the original work is properly cited.