Open Access
ARTICLE
A Lightweight Convolutional Neural Network with Representation Self-challenge for Fingerprint Liveness Detection
1 School of Computer Science, Nanjing University of Information Science and Technology, Nanjing, 210044, China
2Key Laboratory of Public Security Information Application Based on Big-Data Architecture, Ministry of Public Security, Zhejiang Police College, Hangzhou, 310053, China
3 Jiangsu Yuchi Blockchain Research Institute, Nanjing, 210044, China
4 Department of Software Engineering, Lakehead University, Thunder Bay, ON P7B 5E1, Canada
* Corresponding Author: Chengsheng Yuan. Email:
Computers, Materials & Continua 2022, 73(1), 719-733. https://doi.org/10.32604/cmc.2022.027984
Received 30 January 2022; Accepted 30 March 2022; Issue published 18 May 2022
Abstract
Fingerprint identification systems have been widely deployed in many occasions of our daily life. However, together with many advantages, they are still vulnerable to the presentation attack (PA) by some counterfeit fingerprints. To address challenges from PA, fingerprint liveness detection (FLD) technology has been proposed and gradually attracted people's attention. The vast majority of the FLD methods directly employ convolutional neural network (CNN), and rarely pay attention to the problem of over-parameterization and over-fitting of models, resulting in large calculation force of model deployment and poor model generalization. Aiming at filling this gap, this paper designs a lightweight multi-scale convolutional neural network method, and further proposes a novel hybrid spatial pyramid pooling block to extract abundant features, so that the number of model parameters is greatly reduced, and support multi-scale true/fake fingerprint detection. Next, the representation self-challenge (RSC) method is used to train the model, and the attention mechanism is also adopted for optimization during execution, which alleviates the problem of model over-fitting and enhances generalization of detection model. Finally, experimental results on two publicly benchmarks: LivDet2011 and LivDet2013 sets, show that our method achieves outstanding detection results for blind materials and cross-sensor. The size of the model parameters is only 548 KB, and the average detection error of cross-sensors and cross-materials are 15.22 and 1 respectively, reaching the highest level currently available.Keywords
Cite This Article
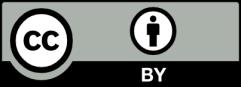
This work is licensed under a Creative Commons Attribution 4.0 International License , which permits unrestricted use, distribution, and reproduction in any medium, provided the original work is properly cited.