Open Access
ARTICLE
Resource Load Prediction of Internet of Vehicles Mobile Cloud Computing
1 School of Computer and Software, Dalian Neusoft University of Information, Dalian, 116023, China
2 School of Information Engineering, Qingdao Bin Hai University, Qingdao, China
3 School of Internet of Things and Software Technology, Wuxi Vocational College of Science and Technology, Wuxi, 214028, China
4 School of Mathematics and Statistics, University College Dublin, Dublin, Ireland
* Corresponding Author: Ning Cao. Email:
Computers, Materials & Continua 2022, 73(1), 165-180. https://doi.org/10.32604/cmc.2022.027776
Received 25 January 2022; Accepted 23 March 2022; Issue published 18 May 2022
Abstract
Load-time series data in mobile cloud computing of Internet of Vehicles (IoV) usually have linear and nonlinear composite characteristics. In order to accurately describe the dynamic change trend of such loads, this study designs a load prediction method by using the resource scheduling model for mobile cloud computing of IoV. Firstly, a chaotic analysis algorithm is implemented to process the load-time series, while some learning samples of load prediction are constructed. Secondly, a support vector machine (SVM) is used to establish a load prediction model, and an improved artificial bee colony (IABC) function is designed to enhance the learning ability of the SVM. Finally, a CloudSim simulation platform is created to select the per-minute CPU load history data in the mobile cloud computing system, which is composed of 50 vehicles as the data set; and a comparison experiment is conducted by using a grey model, a back propagation neural network, a radial basis function (RBF) neural network and a RBF kernel function of SVM. As shown in the experimental results, the prediction accuracy of the method proposed in this study is significantly higher than other models, with a significantly reduced real-time prediction error for resource loading in mobile cloud environments. Compared with single-prediction models, the prediction method proposed can build up multidimensional time series in capturing complex load time series, fit and describe the load change trends, approximate the load time variability more precisely, and deliver strong generalization ability to load prediction models for mobile cloud computing resources.Keywords
Cite This Article
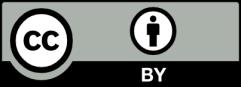
This work is licensed under a Creative Commons Attribution 4.0 International License , which permits unrestricted use, distribution, and reproduction in any medium, provided the original work is properly cited.