Open Access
ARTICLE
Threshold Filtering Semi-Supervised Learning Method for SAR Target Recognition
1 Harbin Engineering University, College of Computer Science and Technology, Harbin, 150001, China
2 Xidian University, Xi’an, 710000, China
3 The 54th Research Institute of CETC, Shijiazhuang, 050000, China
4 University of Bologna, Bologna, 40100, Italy
* Corresponding Author: Ye Tian. Email:
Computers, Materials & Continua 2022, 73(1), 465-476. https://doi.org/10.32604/cmc.2022.027488
Received 19 January 2022; Accepted 01 April 2022; Issue published 18 May 2022
Abstract
The semi-supervised deep learning technology driven by a small part of labeled data and a large amount of unlabeled data has achieved excellent performance in the field of image processing. However, the existing semi-supervised learning techniques are all carried out under the assumption that the labeled data and the unlabeled data are in the same distribution, and its performance is mainly due to the two being in the same distribution state. When there is out-of-class data in unlabeled data, its performance will be affected. In practical applications, it is difficult to ensure that unlabeled data does not contain out-of-category data, especially in the field of Synthetic Aperture Radar (SAR) image recognition. In order to solve the problem that the unlabeled data contains out-of-class data which affects the performance of the model, this paper proposes a semi-supervised learning method of threshold filtering. In the training process, through the two selections of data by the model, unlabeled data outside the category is filtered out to optimize the performance of the model. Experiments were conducted on the Moving and Stationary Target Acquisition and Recognition (MSTAR) dataset, and compared with existing several state-of-the-art semi-supervised classification approaches, the superiority of our method was confirmed, especially when the unlabeled data contained a large amount of out-of-category data.Keywords
Cite This Article
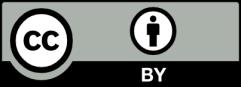
This work is licensed under a Creative Commons Attribution 4.0 International License , which permits unrestricted use, distribution, and reproduction in any medium, provided the original work is properly cited.