Open Access
ARTICLE
Bio-inspired Hybrid Feature Selection Model for Intrusion Detection
1 Computer Science Department, The World Islamic Sciences and Education University, Amman, Jordan
2 Network and Cybersecurity Department, Teesside University, Middlesbrough, United Kingdom
3 Information and Network Security, The World Islamic Sciences and Education University, Amman, Jordan
4 Software Engineering, The World Islamic Sciences and Education University, Amman, Jordan
* Corresponding Author: Adel Hamdan Mohammad. Email:
Computers, Materials & Continua 2022, 73(1), 133-150. https://doi.org/10.32604/cmc.2022.027475
Received 18 January 2022; Accepted 23 March 2022; Issue published 18 May 2022
Abstract
Intrusion detection is a serious and complex problem. Undoubtedly due to a large number of attacks around the world, the concept of intrusion detection has become very important. This research proposes a multilayer bio-inspired feature selection model for intrusion detection using an optimized genetic algorithm. Furthermore, the proposed multilayer model consists of two layers (layers 1 and 2). At layer 1, three algorithms are used for the feature selection. The algorithms used are Particle Swarm Optimization (PSO), Grey Wolf Optimization (GWO), and Firefly Optimization Algorithm (FFA). At the end of layer 1, a priority value will be assigned for each feature set. At layer 2 of the proposed model, the Optimized Genetic Algorithm (GA) is used to select one feature set based on the priority value. Modifications are done on standard GA to perform optimization and to fit the proposed model. The Optimized GA is used in the training phase to assign a priority value for each feature set. Also, the priority values are categorized into three categories: high, medium, and low. Besides, the Optimized GA is used in the testing phase to select a feature set based on its priority. The feature set with a high priority will be given a high priority to be selected. At the end of phase 2, an update for feature set priority may occur based on the selected features priority and the calculated F-Measures. The proposed model can learn and modify feature sets priority, which will be reflected in selecting features. For evaluation purposes, two well-known datasets are used in these experiments. The first dataset is UNSW-NB15, the other dataset is the NSL-KDD. Several evaluation criteria are used, such as precision, recall, and F-Measure. The experiments in this research suggest that the proposed model has a powerful and promising mechanism for the intrusion detection system.Keywords
Cite This Article
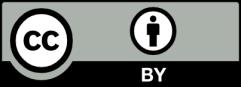
This work is licensed under a Creative Commons Attribution 4.0 International License , which permits unrestricted use, distribution, and reproduction in any medium, provided the original work is properly cited.