Open Access
ARTICLE
Lower-Limb Motion-Based Ankle-Foot Movement Classification Using 2D-CNN
1 School of Telecommunication Engineering, Institute of Engineering, Suranaree University of Technology, Nakhon Ratchasima, 30000, Thailand
2 Department of Telecommunications Engineering, Faculty of Engineering and Technology, Rajamangala University of Technology Isan (RMUTI), Nakhon Ratchasima, 30000, Thailand
3 Orthopedic Department School of Medicine, Suranaree University of Technology, Nakhon Ratchasima, 30000, Thailand
4 School of Mechanical Engineering, Institute of Engineering, Suranaree University of Technology, Nakhon Ratchasima, 30000, Thailand
* Corresponding Author: Peerapong Uthansakul. Email:
Computers, Materials & Continua 2022, 73(1), 1269-1282. https://doi.org/10.32604/cmc.2022.027474
Received 18 January 2022; Accepted 11 April 2022; Issue published 18 May 2022
Abstract
Recently, the Muscle-Computer Interface (MCI) has been extensively popular for employing Electromyography (EMG) signals to help the development of various assistive devices. However, few studies have focused on ankle foot movement classification considering EMG signals at limb position. This work proposes a new framework considering two EMG signals at a lower-limb position to classify the ankle movement characteristics based on normal walking cycles. For this purpose, we introduce a human ankle-foot movement classification method using a two-dimensional-convolutional neural network (2D-CNN) with low-cost EMG sensors based on lower-limb motion. The time-domain signals of EMG obtained from two sensors belonging to Dorsiflexion, Neutral-position, and Plantarflexion are firstly converted into time-frequency spectrograms by short-time Fourier transform. Afterward, the spectrograms of the three ankle-foot movement types are used as input to the 2D-CNN such that the EMG foot movement types are finally classified. For the evaluation phase, the proposed method is investigated using the healthy volunteer for 5-fold cross-validation, and the accuracy is used as a standard evaluation. The results demonstrate that our approach provides an average accuracy of 99.34%. This exhibits the usefulness of 2D-CNN with low-cost EMG sensors in terms of ankle-foot movement classification at limb position, which offers feasibility for walking. However, the obtained EMG signal is not directly considered at the ankle position.Keywords
Cite This Article
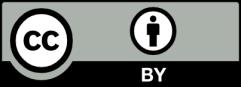
This work is licensed under a Creative Commons Attribution 4.0 International License , which permits unrestricted use, distribution, and reproduction in any medium, provided the original work is properly cited.