Open Access
ARTICLE
CNN-BiLSTM-Attention Model in Forecasting Wave Height over South-East China Seas
1 School of Artificial Intelligence (School of Future Technology), Nanjing University of Information Science and Technology Nanjing, 210044, China
2 Southern Marine Science and Engineering Guangdong Laboratory (Zhuhai), Zhuhai, 519080, China
3 School of Marine Sciences, Nanjing University of Information Science and Technology, Nanjing, 210044, China
4 International Business Machines Corporation (IBM), New York, 10504, USA
* Corresponding Author: Lina Wang. Email:
Computers, Materials & Continua 2022, 73(1), 2151-2168. https://doi.org/10.32604/cmc.2022.027415
Received 18 January 2022; Accepted 19 April 2022; Issue published 18 May 2022
Abstract
Though numerical wave models have been applied widely to significant wave height prediction, they consume massive computing memory and their accuracy needs to be further improved. In this paper, a two-dimensional (2D) significant wave height (SWH) prediction model is established for the South and East China Seas. The proposed model is trained by Wave Watch III (WW3) reanalysis data based on a convolutional neural network, the bi-directional long short-term memory and the attention mechanism (CNN-BiLSTM-Attention). It adopts the convolutional neural network to extract spatial features of original wave height to reduce the redundant information input into the BiLSTM network. Meanwhile, the BiLSTM model is applied to fully extract the features of the associated information of time series data. Besides, the attention mechanism is used to assign probability weight to the output information of the BiLSTM layer units, and finally, a training model is constructed. Up to 24-h prediction experiments are conducted under normal and extreme conditions, respectively. Under the normal wave condition, for 3-, 6-, 12- and 24-h forecasting, the mean values of the correlation coefficients on the test set are 0.996, 0.991, 0.980, and 0.945, respectively. The corresponding mean values of the root mean square errors are measured at 0.063 m, 0.105 m, 0.172 m, and 0.281 m, respectively. Under the typhoon-forced extreme condition, the model based on CNN-BiLSTM-Attention is trained by typhoon-induced SWH extracted from the WW3 reanalysis data. For 3-, 6-, 12- and 24-h forecasting, the mean values of correlation coefficients on the test set are respectively 0.993, 0.983, 0.958, and 0.921, and the averaged root mean square errors are 0.159 m, 0.257 m, 0.437 m, and 0.555 m, respectively. The model performs better than that trained by all the WW3 reanalysis data. The result suggests that the proposed algorithm can be applied to the 2D wave forecast with higher accuracy and efficiency.Keywords
Cite This Article
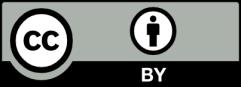
This work is licensed under a Creative Commons Attribution 4.0 International License , which permits unrestricted use, distribution, and reproduction in any medium, provided the original work is properly cited.