Open Access
ARTICLE
Unsupervised Graph-Based Tibetan Multi-Document Summarization
1 School of Information and Engineering, Minzu University of China, Beijing, 100081, China
2 National Language Resource Monitoring & Research Center, Minority Languages Branch, Beijing, 100081, China
3 University of California, Irvine, California, 92617, USA
4 Department of Physics, New Jersey Institute of Technology, Newark, New Jersey, 07102-1982, USA
* Corresponding Author: Wei Song. Email:
Computers, Materials & Continua 2022, 73(1), 1769-1781. https://doi.org/10.32604/cmc.2022.027301
Received 14 January 2022; Accepted 02 April 2022; Issue published 18 May 2022
Abstract
Text summarization creates subset that represents the most important or relevant information in the original content, which effectively reduce information redundancy. Recently neural network method has achieved good results in the task of text summarization both in Chinese and English, but the research of text summarization in low-resource languages is still in the exploratory stage, especially in Tibetan. What’s more, there is no large-scale annotated corpus for text summarization. The lack of dataset severely limits the development of low-resource text summarization. In this case, unsupervised learning approaches are more appealing in low-resource languages as they do not require labeled data. In this paper, we propose an unsupervised graph-based Tibetan multi-document summarization method, which divides a large number of Tibetan news documents into topics and extracts the summarization of each topic. Summarization obtained by using traditional graph-based methods have high redundancy and the division of documents topics are not detailed enough. In terms of topic division, we adopt two level clustering methods converting original document into document-level and sentence-level graph, next we take both linguistic and deep representation into account and integrate external corpus into graph to obtain the sentence semantic clustering. Improve the shortcomings of the traditional K-Means clustering method and perform more detailed clustering of documents. Then model sentence clusters into graphs, finally remeasure sentence nodes based on the topic semantic information and the impact of topic features on sentences, higher topic relevance summary is extracted. In order to promote the development of Tibetan text summarization, and to meet the needs of relevant researchers for high-quality Tibetan text summarization datasets, this paper manually constructs a Tibetan summarization dataset and carries out relevant experiments. The experiment results show that our method can effectively improve the quality of summarization and our method is competitive to previous unsupervised methods.Keywords
Cite This Article
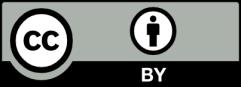
This work is licensed under a Creative Commons Attribution 4.0 International License , which permits unrestricted use, distribution, and reproduction in any medium, provided the original work is properly cited.