Open Access
ARTICLE
A TMA-Seq2seq Network for Multi-Factor Time Series Sea Surface Temperature Prediction
1 Department of Information Technology, Shanghai Ocean University, Shanghai, 201306, China
2 State Key Laboratory of Satellite Ocean Environment Dynamics, Second Institute of Oceanography, Ministry of Natural Resources, Hangzhou, 310012, China
3 College of Computer, Dong Hua University, Shanghai, 200051, China
4 College of Information Technology, Shanghai Jian Qiao University, Shanghai, 201306, China
5 Software Engineering Department, College of Computer and Information Sciences, King Saud University, Riyadh 12372, Saudi Arabia
6 Department of Electrical Engineering, Sangmyung University, South Korea, Korea
* Corresponding Author: Wei Song. Email:
Computers, Materials & Continua 2022, 73(1), 51-67. https://doi.org/10.32604/cmc.2022.026771
Received 04 January 2022; Accepted 02 March 2022; Issue published 18 May 2022
Abstract
Sea surface temperature (SST) is closely related to global climate change, ocean ecosystem, and ocean disaster. Accurate prediction of SST is an urgent and challenging task. With a vast amount of ocean monitoring data are continually collected, data-driven methods for SST time-series prediction show promising results. However, they are limited by neglecting complex interactions between SST and other ocean environmental factors, such as air temperature and wind speed. This paper uses multi-factor time series SST data to propose a sequence-to-sequence network with two-module attention (TMA-Seq2seq) for long-term time series SST prediction. Specifically, TMA-Seq2seq is an LSTM-based encoder-decoder architecture facilitated by factor- and temporal-attention modules and the input of multi-factor time series. It takes six-factor time series as the input, namely air temperature, air pressure, wind speed, wind direction, SST, and SST anomaly (SSTA). A factor attention module is first designed to adaptively learn the effect of different factors on SST, followed by an encoder to extract factor-attention weighted features as feature representations. And then, a temporal attention module is designed to adaptively select the hidden states of the encoder across all time steps to learn more robust temporal relationships. The decoder follows the temporal-attention module to decode the feature vector concatenated from the weighted features and original input feature. Finally, we use a fully-connect layer to map the feature into prediction results. With the two attention modules, our model effectively improves the prediction accuracy of SST since it can not only extract relevant factor features but also boost the long-term dependency. Extensive experiments on the datasets of China Coastal Sites (CCS) demonstrate that our proposed model outperforms other methods, reaching 98.29% in prediction accuracy (PACC) and 0.34 in root mean square error (RMSE). Moreover, SST prediction experiments in China’s East, South, and Yellow Sea site data show that the proposed model has strong robustness and multi-site applicability.Keywords
Cite This Article
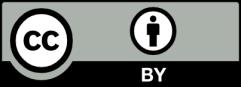