Open Access
ARTICLE
Evolutionary Intelligence and Deep Learning Enabled Diabetic Retinopathy Classification Model
1 MIS Department, College of Business Administration, University of Business and Technology, Jeddah, 21448, Saudi Arabia
2 Department of Computer Science, Faculty of Information Technology, Al-Hussein Bin Talal University, Ma’an, 71111, Jordan
3 School of Engineering, Princess Sumaya University for Technology, Amman, 11941, Jordan.
* Corresponding Author: Bassam A. Y. Alqaralleh. Email:
Computers, Materials & Continua 2022, 73(1), 87-101. https://doi.org/10.32604/cmc.2022.026729
Received 03 January 2022; Accepted 18 March 2022; Issue published 18 May 2022
Abstract
Diabetic Retinopathy (DR) has become a widespread illness among diabetics across the globe. Retinal fundus images are generally used by physicians to detect and classify the stages of DR. Since manual examination of DR images is a time-consuming process with the risks of biased results, automated tools using Artificial Intelligence (AI) to diagnose the disease have become essential. In this view, the current study develops an Optimal Deep Learning-enabled Fusion-based Diabetic Retinopathy Detection and Classification (ODL-FDRDC) technique. The intention of the proposed ODL-FDRDC technique is to identify DR and categorize its different grades using retinal fundus images. In addition, ODL-FDRDC technique involves region growing segmentation technique to determine the infected regions. Moreover, the fusion of two DL models namely, CapsNet and MobileNet is used for feature extraction. Further, the hyperparameter tuning of these models is also performed via Coyote Optimization Algorithm (COA). Gated Recurrent Unit (GRU) is also utilized to identify DR. The experimental results of the analysis, accomplished by ODL-FDRDC technique against benchmark DR dataset, established the supremacy of the technique over existing methodologies under different measures.Keywords
Cite This Article
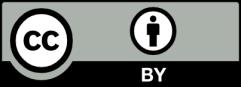
This work is licensed under a Creative Commons Attribution 4.0 International License , which permits unrestricted use, distribution, and reproduction in any medium, provided the original work is properly cited.