Open Access
ARTICLE
Feature Extraction and Classification of Plant Leaf Diseases Using Deep Learning Techniques
1 Department of Electronics and Communication Engineering, Saveetha School of Engineering, Saveetha Institute of Medical and Technical Sciences (SIMATS), Saveetha University, Thandalam, Chennai, 602105, India
2 Instituteof Bio-Medical Engineering, Saveetha School of Engineering, Saveetha Institute of Medical and Technical Sciences (SIMATS), Saveetha University, Thandalam, Chennai, 602105, India
* Corresponding Author: S. Srinivasan. Email:
Computers, Materials & Continua 2022, 73(1), 233-247. https://doi.org/10.32604/cmc.2022.026542
Received 29 December 2021; Accepted 02 March 2022; Issue published 18 May 2022
Abstract
In India’s economy, agriculture has been the most significant contributor. Despite the fact that agriculture’s contribution is decreasing as the world’s population grows, it continues to be the most important source of employment with a little margin of difference. As a result, there is a pressing need to pick up the pace in order to achieve competitive, productive, diverse, and long-term agriculture. Plant disease misinterpretations can result in the incorrect application of pesticides, causing crop harm. As a result, early detection of infections is critical as well as cost-effective for farmers. To diagnose the disease at an earlier stage, appropriate segmentation of the diseased component from the leaf in an accurate manner is critical. However, due to the existence of noise in the digitally captured image, as well as variations in backdrop, shape, and brightness in sick photographs, effective recognition has become a difficult task. Leaf smut, Bacterial blight and Brown spot diseases are segmented and classified using diseased Apple (20), Cercospora (60), Rice (100), Grape (140), and wheat (180) leaf photos in the suggested work. In addition, a superior segmentation technique for the ROI from sick leaves with living backdrop is presented here. Textural features of the segmented ROI, such as 1st and 2nd order WPCA Features, are discovered after segmentation. This comprises 1st order textural features like kurtosis, skewness, mean and variance as well as 2nd procedure textural features like smoothness, energy, correlation, homogeneity, contrast, and entropy. Finally, the segmented region of interest’s textural features is fed into four different classifiers, with the Enhanced Deep Convolutional Neural Network proving to be the most precise, with a 96.1% accuracy.Keywords
Cite This Article
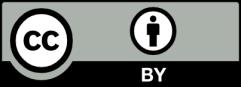
This work is licensed under a Creative Commons Attribution 4.0 International License , which permits unrestricted use, distribution, and reproduction in any medium, provided the original work is properly cited.