Open Access
ARTICLE
A Hybrid Neural Network-based Approach for Forecasting Water Demand
1 Department of Computer Science and Artificial Intelligence, College of Computer Sciences and Engineering, University of Jeddah, Jeddah 21959, Saudi Arabia
2 Department of Computer and Network Engineering, College of Computer Sciences and Engineering, University of Jeddah, Jeddah 21959, Saudi Arabia
3 Department of Information Systems and Technology, College of Computer Sciences and Engineering, University of Jeddah, Jeddah 21959, Saudi Arabia
* Corresponding Author: Al-Batool Al-Ghamdi. Email:
Computers, Materials & Continua 2022, 73(1), 1365-1383. https://doi.org/10.32604/cmc.2022.026246
Received 20 December 2021; Accepted 02 March 2022; Issue published 18 May 2022
Abstract
Water is a vital resource. It supports a multitude of industries, civilizations, and agriculture. However, climatic conditions impact water availability, particularly in desert areas where the temperature is high, and rain is scarce. Therefore, it is crucial to forecast water demand to provide it to sectors either on regular or emergency days. The study aims to develop an accurate model to forecast daily water demand under the impact of climatic conditions. This forecasting is known as a multivariate time series because it uses both the historical data of water demand and climatic conditions to forecast the future. Focusing on the collected data of Jeddah city, Saudi Arabia in the period between 2004 and 2018, we develop a hybrid approach that uses Artificial Neural Networks (ANN) for forecasting and Particle Swarm Optimization algorithm (PSO) for tuning ANNs’ hyperparameters. Based on the Root Mean Square Error (RMSE) metric, results show that the (PSO-ANN) is an accurate model for multivariate time series forecasting. Also, the first day is the most difficult day for prediction (highest error rate), while the second day is the easiest to predict (lowest error rate). Finally, correlation analysis shows that the dew point is the most climatic factor affecting water demand.Keywords
Cite This Article
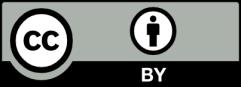