Open Access
ARTICLE
Mutation Prediction for Coronaviruses Using Genome Sequence and Recurrent Neural Networks
1 Bharati Vidyapeeth’s College of Engineering, New Delhi, 110063, India
2 Department of Natural and Exact Sciences, Samarkand State University, Samarkand, Uzbekistan
3 Graduate School, Duy Tan University, Da Nang, 550000, Viet Nam
4 Faculty of Information Technology, Duy Tan University, Da Nang, 550000, Viet Nam
5 Department of Information Technology, College of Computers and Information Technology, Taif University, Taif, 21944, Saudi Arabia
* Corresponding Author: Anand Nayyar. Email:
Computers, Materials & Continua 2022, 73(1), 1601-1619. https://doi.org/10.32604/cmc.2022.026205
Received 18 December 2021; Accepted 08 April 2022; Issue published 18 May 2022
Abstract
The study of viruses and their genetics has been an opportunity as well as a challenge for the scientific community. The recent ongoing SARS-Cov2 (Severe Acute Respiratory Syndrome) pandemic proved the unpreparedness for these situations. Not only the countermeasures for the effect caused by virus need to be tackled but the mutation taking place in the very genome of the virus is needed to be kept in check frequently. One major way to find out more information about such pathogens is by extracting the genetic data of such viruses. Though genetic data of viruses have been cultured and stored as well as isolated in form of their genome sequences, there is still limited methods on what new viruses can be generated in future due to mutation. This research proposes a deep learning model to predict the genome sequences of the SARS-Cov2 virus using only the previous viruses of the coronaviridae family with the help of RNN-LSTM (Recurrent Neural Network-Long Short-Term Memory) and RNN-GRU (Gated Recurrent Unit) so that in the future, several counter measures can be taken by predicting possible changes in the genome with the help of existing mutations in the virus. After the process of testing the model, the F1-recall came out to be more than 0.95. The mutation detection’s accuracy of both the models come out about 98.5% which shows the capability of the recurrent neural network to predict future changes in the genome of virus.Keywords
Cite This Article
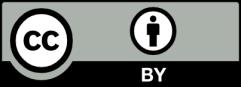
This work is licensed under a Creative Commons Attribution 4.0 International License , which permits unrestricted use, distribution, and reproduction in any medium, provided the original work is properly cited.