Open Access
ARTICLE
An Optimal Framework for SDN Based on Deep Neural Network
1 Department of Industrial Engineering, School of Applied Technical Sciences German Jordanian University, Amman, 35247, Jordan
2 School of Electrical and Electronic Engineering, Universiti Sains Malaysia, Nibong Tebal, 14300, Malaysia
3 Center for Artificial Intelligence Technology, Faculty of Information Science and Technology, The National University of Malaysia (UKM), Bangi, 43600, Selangor, Malaysia
4 Department of Electrical Engineering, University of Engineering and Technology, Peshawar, 814, Pakistan
5 Turabah University College, Computer Sciences Program, Taif University, Taif, 21944, Saudi Arabia
6 Research Institute for Information Technology, Kyushu University, Fukuoka, 819-0395, Japan
7 Computer Science-Mathematics Department, Faculty of Science, South Valley University, Qena, 83523, Egypt
* Corresponding Author: Samih M. Mostafa. Email:
Computers, Materials & Continua 2022, 73(1), 1125-1140. https://doi.org/10.32604/cmc.2022.025810
Received 05 December 2021; Accepted 12 January 2022; Issue published 18 May 2022
Abstract
Software-defined networking (SDN) is a new paradigm that promises to change by breaking vertical integration, decoupling network control logic from the underlying routers and switches, promoting (logical) network control centralization, and introducing network programming. However, the controller is similarly vulnerable to a “single point of failure”, an attacker can execute a distributed denial of service (DDoS) attack that invalidates the controller and compromises the network security in SDN. To address the problem of DDoS traffic detection in SDN, a novel detection approach based on information entropy and deep neural network (DNN) is proposed. This approach contains a DNN-based DDoS traffic detection module and an information-based entropy initial inspection module. The initial inspection module detects the suspicious network traffic by computing the information entropy value of the data packet's source and destination Internet Protocol (IP) addresses, and then identifies it using the DDoS detection module based on DNN. DDoS assaults were found when suspected irregular traffic was validated. Experiments reveal that the algorithm recognizes DDoS activity at a rate of more than 99%, with a much better accuracy rate. The false alarm rate (FAR) is much lower than that of the information entropy-based detection method. Simultaneously, the proposed framework can shorten the detection time and improve the resource utilization efficiency.Keywords
Cite This Article
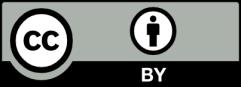