Open Access
ARTICLE
Deep Learning Enabled Disease Diagnosis for Secure Internet of Medical Things
1 Department of Computer Science, College of Computer Engineering and Sciences, Prince Sattam Bin Abdulaziz University, Alkharj, 11942, Saudi Arabia
2 College of Computer and Information Sciences, Imam Mohammad Ibn Saud Islamic University (IMSIU), Riyadh, 11432, Saudi Arabia
3 College of Pharmacy, King Saud University, Riyadh, 11451, Saudi Arabia
4 Department of Computer Science and Information System, College of Applied Sciences, AlMaarefa University, Riyadh, 11597, Saudi Arabia
5 Department of Information Technology, FPT University, Ho Chi Minh City, Vietnam
6 Department of Computer Science and Engineering, Sejong University, Seoul, 05006, Korea
* Corresponding Author: Hyeonjoon Moon. Email:
Computers, Materials & Continua 2022, 73(1), 965-979. https://doi.org/10.32604/cmc.2022.025760
Received 03 December 2021; Accepted 02 March 2022; Issue published 18 May 2022
Abstract
In recent times, Internet of Medical Things (IoMT) gained much attention in medical services and healthcare management domain. Since healthcare sector generates massive volumes of data like personal details, historical medical data, hospitalization records, and discharging records, IoMT devices too evolved with potentials to handle such high quantities of data. Privacy and security of the data, gathered by IoMT gadgets, are major issues while transmitting or saving it in cloud. The advancements made in Artificial Intelligence (AI) and encryption techniques find a way to handle massive quantities of medical data and achieve security. In this view, the current study presents a new Optimal Privacy Preserving and Deep Learning (DL)-based Disease Diagnosis (OPPDL-DD) in IoMT environment. Initially, the proposed model enables IoMT devices to collect patient data which is then preprocessed to optimize quality. In order to decrease the computational difficulty during diagnosis, Radix Tree structure is employed. In addition, ElGamal public key cryptosystem with Rat Swarm Optimizer (EIG-RSO) is applied to encrypt the data. Upon the transmission of encrypted data to cloud, respective decryption process occurs and the actual data gets reconstructed. Finally, a hybridized methodology combining Gated Recurrent Unit (GRU) with Convolution Neural Network (CNN) is exploited as a classification model to diagnose the disease. Extensive sets of simulations were conducted to highlight the performance of the proposed model on benchmark dataset. The experimental outcomes ensure that the proposed model is superior to existing methods under different measures.Keywords
Cite This Article
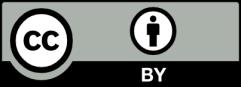
This work is licensed under a Creative Commons Attribution 4.0 International License , which permits unrestricted use, distribution, and reproduction in any medium, provided the original work is properly cited.