Open Access
ARTICLE
Pulmonary Diseases Decision Support System Using Deep Learning Approach
1 Department of Computer Engineering, Yarmouk University, Irbid, 21163, Jordan
2 Department of Biomedical Systems and Informatics Engineering, Yarmouk University, Irbid, 21163, Jordan
3 Department of Biomedical Engineering, Jordan University of Science and Technology, Irbid 22110, Jordan
4 Pediatrician, Mediclinic Hospital, Al Ain, 14444, UAE
* Corresponding Author: Ali Mohammad Alqudah. Email:
Computers, Materials & Continua 2022, 73(1), 311-326. https://doi.org/10.32604/cmc.2022.025750
Received 03 December 2021; Accepted 17 March 2022; Issue published 18 May 2022
Abstract
Pulmonary diseases are common throughout the world, especially in developing countries. These diseases include chronic obstructive pulmonary diseases, pneumonia, asthma, tuberculosis, fibrosis, and recently COVID-19. In general, pulmonary diseases have a similar footprint on chest radiographs which makes them difficult to discriminate even for expert radiologists. In recent years, many image processing techniques and artificial intelligence models have been developed to quickly and accurately diagnose lung diseases. In this paper, the performance of four popular pretrained models (namely VGG16, DenseNet201, DarkNet19, and XceptionNet) in distinguishing between different pulmonary diseases was analyzed. To the best of our knowledge, this is the first published study to ever attempt to distinguish all four cases normal, pneumonia, COVID-19 and lung opacity from Chest-X-Ray (CXR) images. All models were trained using Chest-X-Ray (CXR) images, and statistically tested using 5-fold cross validation. Using individual models, XceptionNet outperformed all other models with a 94.775% accuracy and Area Under the Curve (AUC) of Receiver Operating Characteristic (ROC) of 99.84%. On the other hand, DarkNet19 represents a good compromise between accuracy, fast convergence, resource utilization, and near real time detection (0.33 s). Using a collection of models, the 97.79% accuracy achieved by Ensemble Features was the highest among all surveyed methods, but it takes the longest time to predict an image (5.68 s). An efficient effective decision support system can be developed using one of those approaches to assist radiologists in the field make the right assessment in terms of accuracy and prediction time, such a dependable system can be used in rural areas and various healthcare sectors.Keywords
Cite This Article
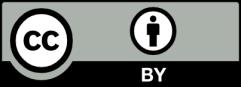
This work is licensed under a Creative Commons Attribution 4.0 International License , which permits unrestricted use, distribution, and reproduction in any medium, provided the original work is properly cited.