Open Access
ARTICLE
Multiple Forgery Detection in Video Using Convolution Neural Network
1 Centre for Advanced Studies, Dr. A P J Abdul Kalam Technical University, Lucknow, India
2 Institute of Engineering and Technology, Dr. A P J Abdul Kalam Technical University, Lucknow, India
* Corresponding Author: Vinay Kumar. Email:
Computers, Materials & Continua 2022, 73(1), 1347-1364. https://doi.org/10.32604/cmc.2022.023545
Received 12 September 2021; Accepted 16 November 2021; Issue published 18 May 2022
Abstract
With the growth of digital media data manipulation in today’s era due to the availability of readily handy tampering software, the authenticity of records is at high risk, especially in video. There is a dire need to detect such problem and do the necessary actions. In this work, we propose an approach to detect the interframe video forgery utilizing the deep features obtained from the parallel deep neural network model and thorough analytical computations. The proposed approach only uses the deep features extracted from the CNN model and then applies the conventional mathematical approach to these features to find the forgery in the video. This work calculates the correlation coefficient from the deep features of the adjacent frames rather than calculating directly from the frames. We divide the procedure of forgery detection into two phases–video forgery detection and video forgery classification. In video forgery detection, this approach detect input video is original or tampered. If the video is not original, then the video is checked in the next phase, which is video forgery classification. In the video forgery classification, method review the forged video for insertion forgery, deletion forgery, and also again check for originality. The proposed work is generalized and it is tested on two different datasets. The experimental results of our proposed model show that our approach can detect the forgery with the accuracy of 91% on VIFFD dataset, 90% in TDTV dataset and classify the type of forgery–insertion and deletion with the accuracy of 82% on VIFFD dataset, 86% on TDTV dataset. This work can helps in the analysis of original and tempered video in various domain.Keywords
Cite This Article
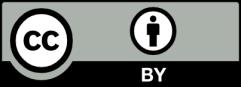
This work is licensed under a Creative Commons Attribution 4.0 International License , which permits unrestricted use, distribution, and reproduction in any medium, provided the original work is properly cited.