Open Access
ARTICLE
An Optimized Neural Network with Bat Algorithm for DNA Sequence Classification
1 Faculty of Computing and Information Technology, Sohar University, Sohar, 311, Sultanate of Oman
2 Soft Computing & Data Mining Centre (SMC), Faculty of Computer Science & Information Technology, Universiti Tun Hussein Onn Malaysia (UTHM), Batu Pahat, 86400, Malaysia
3 School of Electronics, Computing and Mathematics, University of Derby, Derby, DE22 1GB, United Kingdom
4 Institute of Computer Sciences and Information Technology, The University of Agriculture, 25120, Peshawar, Pakistan
5 College of Computing and Informatics, Saudi Electronic University, Riyadh, Saudi Arabia
* Corresponding Author: Muhammad Aamir. Email:
Computers, Materials & Continua 2022, 73(1), 493-511. https://doi.org/10.32604/cmc.2022.021787
Received 14 July 2021; Accepted 30 September 2021; Issue published 18 May 2022
Abstract
Recently, many researchers have used nature inspired metaheuristic algorithms due to their ability to perform optimally on complex problems. To solve problems in a simple way, in the recent era bat algorithm has become famous due to its high tendency towards convergence to the global optimum most of the time. But, still the standard bat with random walk has a problem of getting stuck in local minima. In order to solve this problem, this research proposed bat algorithm with levy flight random walk. Then, the proposed Bat with Levy flight algorithm is further hybridized with three different variants of ANN. The proposed BatLFBP is applied to the problem of insulin DNA sequence classification of healthy homosapien. For classification performance, the proposed models such as Bat levy flight Artificial Neural Network (BatLFANN) and Bat levy Flight Back Propagation (BatLFBP) are compared with the other state-of-the-art algorithms like Bat Artificial Neural Network (BatANN), Bat back propagation (BatBP), Bat Gaussian distribution Artificial Neural Network (BatGDANN). And Bat Gaussian distribution back propagation (BatGDBP), in-terms of means squared error (MSE) and accuracy. From the perspective of simulations results, it is show that the proposed BatLFANN achieved 99.88153% accuracy with MSE of 0.001185, and BatLFBP achieved 99.834185 accuracy with MSE of 0.001658 on WL5. While on WL10 the proposed BatLFANN achieved 99.89899% accuracy with MSE of 0.00101, and BatLFBP achieved 99.84473% accuracy with MSE of 0.004553. Similarly, on WL15 the proposed BatLFANN achieved 99.82853% accuracy with MSE of 0.001715, and BatLFBP achieved 99.3262% accuracy with MSE of 0.006738 which achieve better accuracy as compared to the other hybrid models.
Keywords
Cite This Article
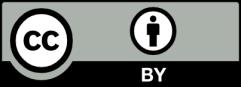
This work is licensed under a Creative Commons Attribution 4.0 International License , which permits unrestricted use, distribution, and reproduction in any medium, provided the original work is properly cited.